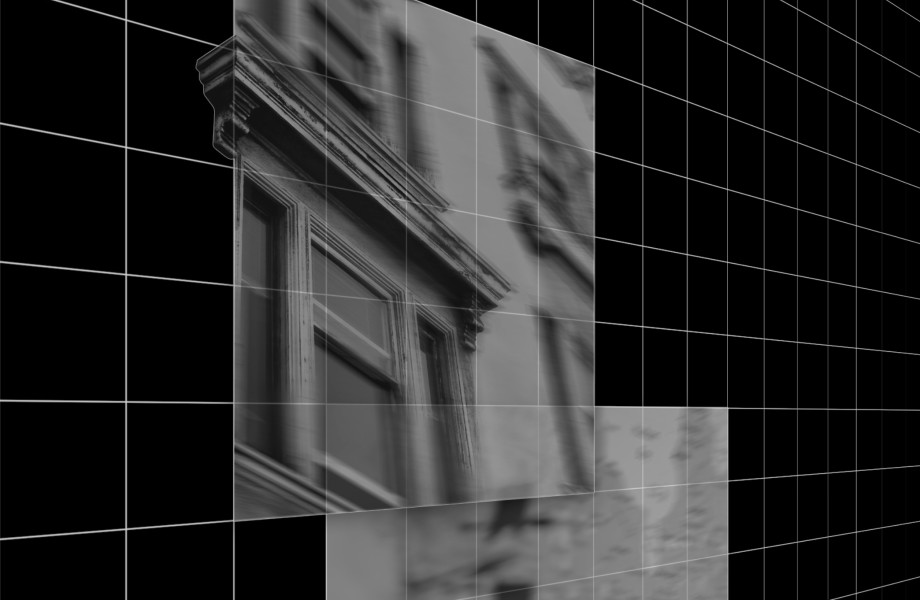
Linear Single- and Three-Phase Voltage Forecasting and Bayesian State Estimation with Limited Sensing
May 22, 2020
Abstract:
Implementing state estimation in low and mediumvoltage power distribution is still challenging given the scale of many networks and the reliance of traditional methods on a large number of measurements. This paper proposes a method to improve voltage predictions in real-time by leveraging a limited set of real-time measurements. The method relies on Bayesian estimation formulated as a linear least squares estimation problem, which resembles the classical weighted least-squares (WLS) approach for scenarios where full network observability is not available. We build on recently developed linear approximations for unbalanced three-phase power flow to construct voltage predictions as a linear mapping of load predictions constructed with Gaussian processes. The estimation step to update the voltage forecasts in real-time is a linear computation allowing fast high-resolution state estimate updates. The uncertainty in forecasts can be determined a priori and smoothed a posteriori, making the method useful for both planning, operation and post-hoc analysis. The method outperforms conventional WLS and is applied to different test feeders and validated on a real test feeder with the utility Alliander in The Netherlands.
For full article, head here.
R. Dobbe, W. Van Westering, S. X. Liu, D. Arnold, D. S. Callaway and C. Tomlin, “Linear Single- and Three-Phase Voltage Forecasting and Bayesian State Estimation with Limited Sensing,” in IEEE Transactions on Power Systems.