Illustration by Somnath Bhatt
AI/ML models are curiously everywhere and nowhere.
A guest post by Yung Au. Yung is a doctoral researcher at the Oxford Internet Institute (OII) and a Clarendon Scholar. Her research explores (re)coloniality of tech, surveillance, and verticalities. Twitter: @a_yung_
This essay is part of our ongoing “AI Lexicon” project, a call for contributions to generate alternate narratives, positionalities, and understandings to the better known and widely circulated ways of talking about AI.
AI/ML models are often exported. For example, large tech companies tend to congregate in particular parts of the world and sell software-as-a-service, platform-as-a-service, even surveillance-as-a-service in neatly-bound packages on a subscription basis to individuals, companies, and authorities around the world [1]. As a service/product/labour, AI/ML systems are also frequently exceptionalized where sleek models are intentionally portrayed to magically appear from thin air, skipping the commodity chain altogether. Software and virtual products are often decoupled from their material entanglements — divorced from the vast lithium farms of the Atacama Desert, the cold data centers underneath the Alps, the data annotation centers scattered across the world, and the digital graveyards in the Korle Lagoon [2], [3].
As a feature of our capitalist society, all global supply chains have hidden components — whether it is the obfuscation of sweatshops that operate on child labor or the efforts made towards washing the blood off of the diamond industry. Much important work focuses on unearthing and redressing the unethical operations that undergird the commodities we rely on — and the same applies to data systems. The apparent immateriality of data and its infrastructures however, add an unintuitive dimension to this project. With more physically tangible exports, like a motor engine, we can more intuitively grasp and communicate that the multiple components that constitute it are designed, assembled, and tested in disparate places. A growing body of literature tackles exactly this, to work against this intangibility of data and tying it to its materiality and redressing the injustices left in the wake of AI/ML system development [4]–[6].
AI/ML systems are peculiar exports in another way. They are never quite finished in the same way that many other exports are, such as freshly bound books or fruits that ripen on arrival. AI/ML systems are exported unfinished, with room to tinker and improve. They are trained and tested in lab settings and then trained and tested again in real world settings — constantly learning on the way — a key feature of the iterative process. The decision of when a system is “ready” for deployment is often difficult to ascertain and sometimes decided based on evaluation metrics or arbitrary comparison to some baseline, other times based on contextual needs and urgency.
Where is AI Made?
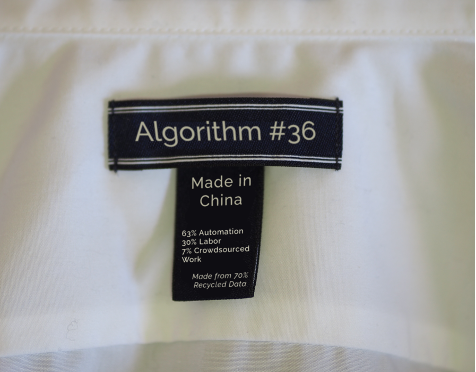
The advent of the Internet has seen the rise of “Anything-as-a-Service” which are services that simplify complex programs into plug-and-play systems where user-friendly endpoints are provided for customers to interface with. This can include anything from commercial spyware systems that provide easy-to-use navigation platforms to intercept large amounts of communications, to facial analysis systems that provide a slick interface that supposedly helps deconstruct one’s emotional states — the list is endless[1]. The provider offers continued maintenance, updates, and troubleshooting support where the client does not necessarily need to have technical knowledge of the systems at hand, how it runs, or what data has trained it.
While such systems may appear simple at the user level, the assembly line of AI systems are long and convoluted. Labor, decisions, and expertise are needed at each stage prior to a model’s deployment, and even still after it is deployed. There is however usually a particular point in this process where a model is deemed to be “made” — a stage where the labor is deemed as most important and valued. With Software-as-a-Service, this is usually the end stages of the product’s life cycle where “intelligence” is offered as a slick package, usually “assembled” at the company’s headquarters, such as California’s Silicon Valley or Dublin’s Silicon Docks. It is at this juncture where code and data are assembled to be intelligent and then exported.
This is despite how many vital components that constitute a system exists before this point. Sareeta Amrute and Luis Felipe Murillo in particular have written about the importance of destabilizing the presumed centers of computing such as Silicon Valley, and instead, redirecting our starting point elsewhere, such as in the geographies that have long been associated with the “South” [7]. This includes highlighting the concerted efforts of data engineers, data labelers, data cleaners that live beyond Silicon Valley for instance [5], [8], [9]. Other scholars, such as Rida Qadri and Nina Dewi Toft Djanegara, have also demonstrated the need to look beyond formally employed data workers and towards the host of human intervention needed for these systems to work — whether it is the local knowledge of drivers that makes food delivery platforms functional, or the expertise of passport photo studio operators that make visual data legible to machines [10], [11].
We, too, are also often part of AI/ML systems, even if we do not know it. We could be part of a training model, such as the Google reCAPTCHA “human intelligence task” systems which prompt users to verify that they are human by typing words as seen from an image which would ultimately help Google Books, Google Street View, Google Search and other services to detect text and numbers automatically [12]. We can also be part of the training after deployment, stuck as part of the endless feedback loop where some communities in particular are deemed as testing grounds for technologies [13], [14].
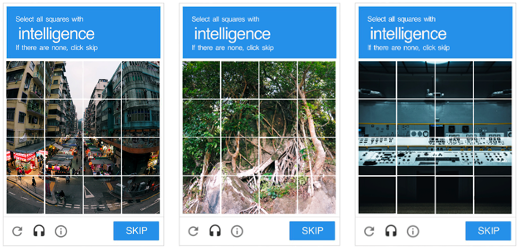
So where is an AI made? What intelligence are we exporting? A disembeded, robotic, metallic intelligence — the intelligence of a few — the intelligence of a thousand people?
AI systems are software and data, but are tied to hardware, and rely on servers, clouds, wires, and people who are simultaneously clients, workers, end-users and data points. Sareeta Amrute and Luis Felipe Murillo highlight the work by post/de-colonial scholarship to examine the obscured labour of computing worlds which are often grouped under the heading of “outsourcing.” This literature examines how the least lucrative jobs in computing are siphoned overseas to peripheral communities (e.g. data labelling and content moderation work). Likewise, the flipside is also deserving of scrutiny: when Western centres of power are the targets of “outsourcing” and when the South becomes reliant on technological services from the West. These under-regulated flows become another way in which imperial dependencies and logics are further entrenched in our systems. AI/ML systems have been touted as a disruption, but in truth, these systems often sit snugly as part of the long, uncomfortable histories of exports and global commodity chains — including the continued patterns of inequitable value extraction and the export of certain ways of doing things.
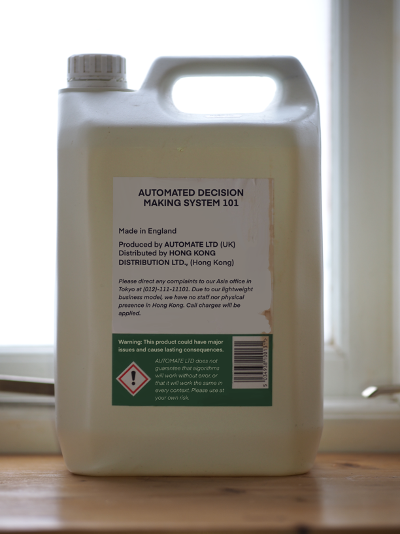
What jurisdictions are AI tethered to?
While intelligence might be everywhere in the process, the power to frame, gate-keep and benefit off of production of AI/ML systems is less distributed. This includes who owns the various data pipelines and data infrastructures at large. Likewise, it matters which jurisdiction these technologies are governed by and which citizens they are accountable to. For example, where are the complaint procedures and warranties bound to? Whose knowledge, needs, and assumptions are prioritized? Just because intelligence is sourced from all stages of production does not mean power is equitably distributed. While platforms and systems might have a vast user-base and worker-base, the number of people who can, in reality, hold a technology giant accountable — to have their concerns heard and acted on — is far fewer [15], [16].
How do we label AI?
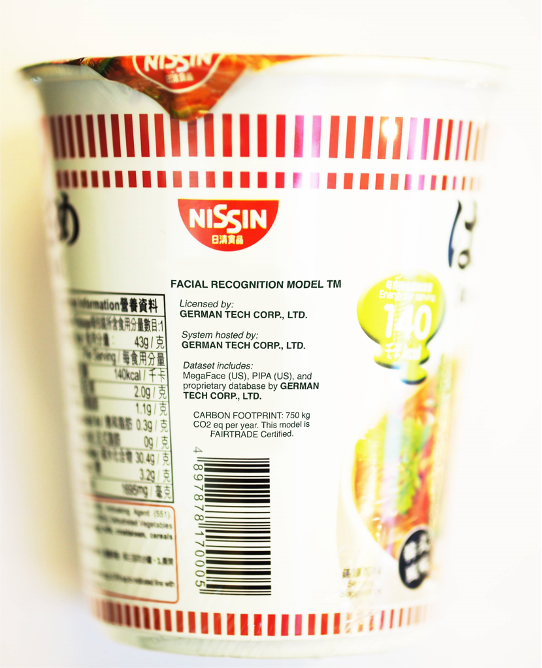
How then, do you label such an elusive, intangible export to truly capture its planetary production process? AI/ML systems appears ill-fitted to many of the categories we are used to thinking through. We are familiar with certain labels, regulations, ways of appraising exports — such as “made in” labels, environmental certifications, ingredients list, or some indication of where the factory that assembled the product is located. We have some intuition as to how to trace the likely source of a faulty engine part or a crate of wine that is not quite up to standard. We can find out which publisher to call up when we find inaccuracies in a manuscript. This is not to say other exports are not problematic or that labels are in any way perfect (for instance, see critiques of Fairtrade standards[1]). Nevertheless, it appears increasingly important to explicate the supply chains in our data systems. How do we assess whether an AI/ML system or its end products uses fair labor, to which jurisdiction it is accountable to, or what their environmental effects are?
Although, like other exports, it is important that our labels, categorizations and imaginaries elucidate rather than obscure. Carbon footprint labelling are becoming more common as we are forced to contend with the ecological consequences of large scale AI/ML systems² [17]. An example ML carbon footprint calculator is³:

Carbon footprints are discussed alongside “carbon offset” — ways to compensate for carbon footprint by funding carbon reduction projects in a distant elsewhere — but of course, the real consequences are rarely that neat. For particularly large-scale projects and products that incorporate AI/ML, the cost of processing power is only a fraction of the environmental impact that is incurred. The sprawling and cascading environmental effects include the carbon emissions and environmental effects incurred from the larger supply and logistic chains that bring these products together, such as hardware and transcontinental infrastructures. These often have very specific requirements, such as the need for vast data centers that can cool off without being too costly, the strategic geographic routes needed for edge computing, the need for materials such as lithium and rare earth metals, and the particular disposal processes of e-waste in the afterlife of the computing industry [2], [18], [21] — all of which contribute to the larger effects of extraction, displacement, and destruction that are almost always felt more acutely by certain communities.
Exporting AI?
Data artefacts are very much deeply entangled with their material basis as a growing body of literature has made abundantly clear. We have gone beyond merely acknowledging the importance of place and have embarked on the difficult project of mapping out exactly the ways that AI/ML systems are tethered to space, time, and matter — as well as its myriad implications. Place matters, including:
- Where an AI/ML model is made; who funds it, who made it and who it is made for
- What data it relies on, who sorts the data and to which categories, whose expertise is used and whose is ignored
- Who has ownership over the data pipelines and computing infrastructure
- Which jurisdiction are these models accountable to
- What raw materials, resources, and labour are needed for the system to be made and be functional
- What are the environmental impacts of the wider supply chain
- What imperial/colonial relations and dependencies are reified in the process
It becomes increasingly important then, to converse about ways to better embed geographies in discussions on AI/ML and its governance. As AI systems maintain their abstract, frictionless, and intangible appearances, they manage to squirm out of regulation, scrutiny, criticism. How can we ground AI, cloud computing, platforms and other lofty corporate-derived words into more tangible and physical terms [6], [22]–[24]? If the infrastructures, imperial legacies, and material benefits are all tangible and geographically situated, how can our vocabularies better reflect this?
What exactly are we exporting when we export AI systems?
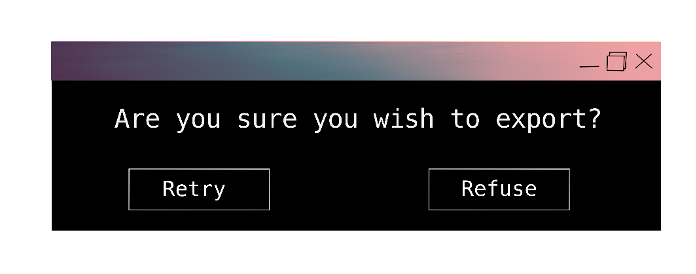
Many thanks to Srujana Katta and Luke Strathmann for their invaluable feedback on the piece.
Footnotes:
[1] These critiques are far ranging, including the problems that emerge from the exclusivity of this particular coalition to the inconsistencies in monitoring standards across markets and across geographic areas
[2] For instance, the world’s largest language model, OpenAI’s GPT-3, referred to as one of the priciest deep learning model, costing over 4.6 million USD and 355 years in computing time
[3] See https://mlco2.github.io/impact/ an example of a simple calculator for the CO2 impact of machine learning models; carbon footprint refers to the Greenhouse Gas (GHG) emissions produced during a process of the production of an item (measured through CO2eq, Carbon Dioxide Equivalent)
References
[1] Y. Au, “Surveillance as a Service,” Oxford, UK: Oxford Commission on AI & Good Governance, 2021.4, 2021.
[2] M. Arboleda, Planetary mine: Territories of extraction under late capitalism. Verso Trade, 2020.
[3] G. A. Akese, “Electronic waste (e-waste) science and advocacy at Agbogbloshie: the making and effects of” The world’s largest e-waste dump”,” PhD Thesis, Memorial University of Newfoundland, 2019.
[4] L. Parks and N. Starosielski, Eds., Signal traffic: critical studies of media infrastructures. Urbana: University of Illinois Press, 2015.
[5] N. Raval, “Interrupting invisibility in a global world,” interactions, vol. 28, no. 4, pp. 27–31, Jun. 2021, doi: 10.1145/3469257.
[6] T.-H. Hu, A Prehistory of the Cloud. MIT press, 2015.
[7] S. Amrute and L. F. R. Murillo, “Introduction: Computing in/from the South,” Catalyst: Feminism, Theory, Technoscience, vol. 6, no. 2, 2020.
[8] M. L. Gray and S. Suri, Ghost Work: How to Stop Silicon Valley from Building a New Global Underclass. Houghton Mifflin Harcourt, 2019.
[9] M. Miceli and J. Posada, “Wisdom for the Crowd: Discoursive Power in Annotation Instructions for Computer Vision,” arXiv:2105.10990 [cs], May 2021, Accessed: Oct. 25, 2021. [Online]. Available: http://arxiv.org/abs/2105.10990
[10] R. Qadri, “Platform workers as infrastructures of global technologies,” interactions, vol. 28, no. 4, pp. 32–35, Jul. 2021, doi: 10.1145/3466162.
[11] N. D. T. Djanegara, “Passport photography and the hidden labor of facial recognition evaluation,” presented at the The 24th ACM Conference on Computer-Supported Cooperative Work and Social Computing, 2021. [Online]. Available: https://cscw.acm.org/2021/
[12] A. A. Casilli, “No CAPTCHA: yet another ruse devised by Google to make you train their robots for free,” Medium, Dec. 06, 2014. https://medium.com/@AntonioCasilli/is-nocaptcha-a-ruse-devised-by-google-to-make-you-work-for-free-for-their-face-recognition-20a7e6a9f700 (accessed Oct. 24, 2021).
[13] P. Arora, “Decolonizing Privacy Studies,” Television & New Media, vol. 20, no. 4, pp. 366–378, May 2019, doi: 10.1177/1527476418806092.
[14] V. Marda and S. Ahmed, “Emotional Entanglement: China’s emotion recognition market and its implications for human rights,” Article 19, 2021.
[15] C. Arun, “Facebook’s Faces,” Social Science Research Network, Rochester, NY, SSRN Scholarly Paper ID 3805210, Mar. 2021. doi: 10.2139/ssrn.3805210.
[16] J. Cowls, M.-T. Png, and Y. Au, “Some Tentative Foundations for ‘Global’ Algorithmic Ethics,” Social Science Research Network, Rochester, NY, SSRN Scholarly Paper ID 3894979, Feb. 2019. Accessed: Aug. 11, 2021. [Online]. Available: https://papers.ssrn.com/abstract=3894979
[17] E. M. Bender, T. Gebru, A. McMillan-Major, and S. Shmitchell, “On the Dangers of Stochastic Parrots: Can Language Models Be Too Big?,” in Proceedings of the 2021 ACM Conference on Fairness, Accountability, and Transparency, New York, NY, USA, Mar. 2021, pp. 610–623. doi: 10.1145/3442188.3445922.
[18] R. Dobbe and M. Whittaker, “AI and Climate Change: How they’re connected, and what we can do about it,” Medium, Oct. 17, 2019. https://medium.com/@AINowInstitute/ai-and-climate-change-how-theyre-connected-and-what-we-can-do-about-it-6aa8d0f5b32c (accessed Oct. 25, 2021).
[19] M. Liboiron, Pollution Is Colonialism. Duke University Press, 2021. doi: 10.1515/9781478021445.
[20] K. Crawford and V. Joler, “Anatomy of an AI System,” Retrieved September, 2018. http://www.anatomyof.ai
[21] Tech Workers Coalition, “Climate Strike,” Tech Workers Coalition, 2019. https://techworkerscoalition.org/climate-strike/ (accessed Oct. 25, 2021).
[22] H. Tawil-Souri, “It’s Still About the Power of Place,” Middle East Journal of Culture and Communication, vol. 5, no. 1, pp. 86–95, Jan. 2012, doi: 10.1163/187398612X624418.
[23] S. L. Star, “The ethnography of infrastructure,” American behavioral scientist, vol. 43, no. 3, pp. 377–391, 1999.
[24] T. Gillespie, Custodians of the Internet: Platforms, content moderation, and the hidden decisions that shape social media. Yale University Press, 2018.