AI Now 2017 Symposium: The Year In Review
The AI Now Initiative recently hosted its annual Symposium at the MIT Media Lab, gathering experts from different disciplines to discuss the social implications of artificial intelligence technologies.
AI Now co-founders Kate Crawford and Meredith Whittaker introduced the event with an opening talk — which you can read below. They drew on examples from the last twelve months to show how quickly AI systems are starting to impact core social institutions.
The Symposium addressed three themes: the state of research (with a focus on bias in machine learning), the state of policy (how will policies related to AI develop in the era of Trump), and the state of law (with a focus on basic rights and liberties). You can watch the full event here.
***
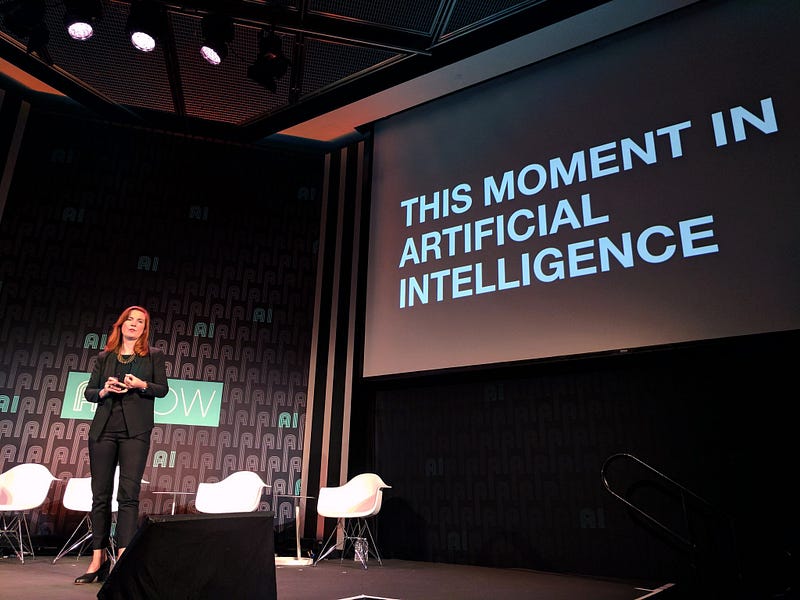
How is artificial intelligence becoming part of our lives? That’s the guiding question for tonight. Artificial intelligence is no longer the dream of distant futures, but is built into banal back-end systems, and embedded in core social institutions. AI systems and related technologies are having an influence on everything from the news you read, to who is released from jail. And the social implications of this shift are not yet fully understood.
But with a term as over-hyped as “artificial intelligence”, we need to ask what it means in the current moment.
As you know, AI has a long history, and its meaning has changed every few years: as technical approaches changed, as funding shifted, and as corporate marketing got involved.
Back in 1956, a small group of men met at Dartmouth College with the aim of creating intelligent machines. They expected to make “significant progress” if they worked on it over a single summer. That might have been somewhat ambitious.
In the last 60 years, the field has progressed in fits and starts, with both big leaps and dead ends. But three developments have greatly accelerated progress in the last decade: cheap computational power, large amounts of data, and better algorithms.
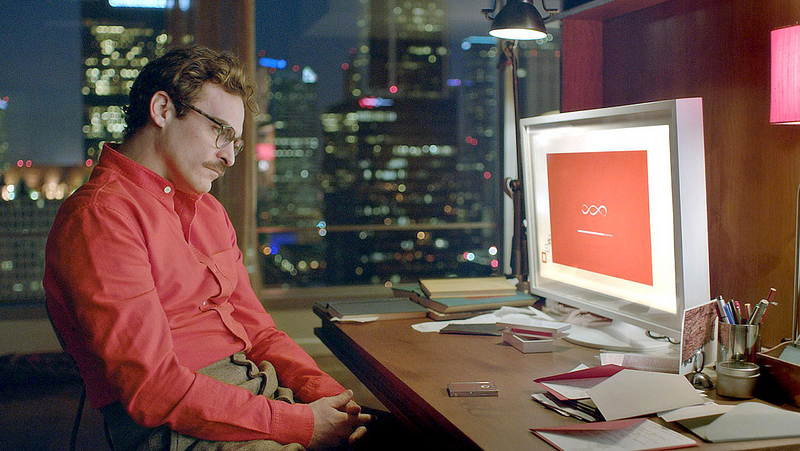
So when people use the term AI today, they’re talking about a grab-bag of techniques, from machine learning to natural language processing to neural networks and beyond. These approaches “learn” about the world by being fed vast amounts of data. So if you saw the movie Her, you might remember that the AI system builds a model of its owner’s personality by “reading” all of his email.
Now imagine AI systems learning from massive troves of Facebook trolling, or Stop-and-Frisk records, with all those skews and prejudices intact.
So for the next decade of AI development, we face challenges that go far beyond the technical: they implicate our core legal, economic and social frameworks.
To provide an introduction, we are going to give you a high-speed tour through the topics of the night — bias, policy gaps, and rights and liberties. And, we’re only going to use examples from the past year to provide a visceral sense of how rapidly the social impacts of AI are emerging.
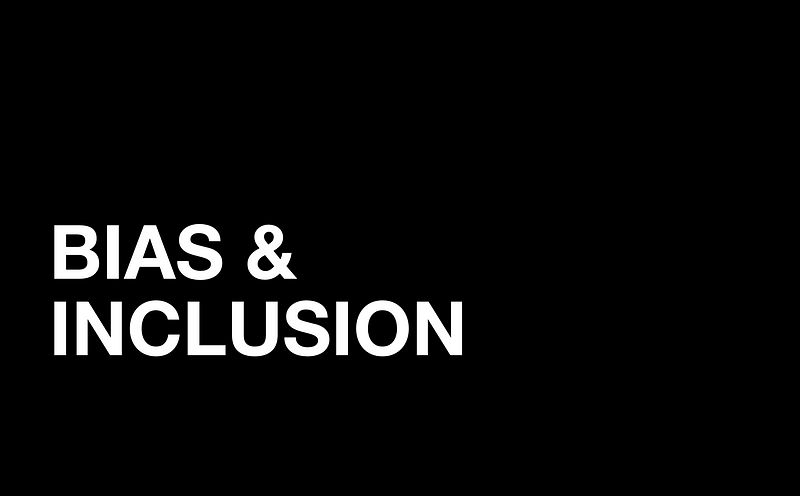
The first topic of the night looks at Bias and Inclusion. There has been a big spike of research in this area very recently, supported by research communities like the one at the Fairness, Accountability, and Transparency in Machine Learning Workshop.
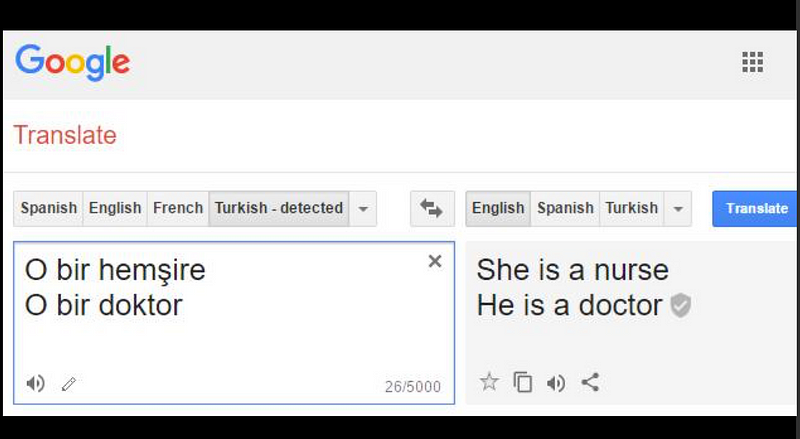
Recent papers have shown gender biases embedded in the models that do natural language processing. Researchers have shown how NLP models create stereotypical associations, like “woman” with “nurse” and “man” with “doctor”.
But while we’ve seen more computer scientists address issues of fairness and bias, there are big disagreements about what to do about it — and whether bias can ever be truly erased. Human data is embedded in human culture, and models are shaped by the corpora that they train on — be that the dataset of militarized interstate disputes, fashion photography, UFO sightings, or the Enron emails. So “scrubbing them to neutral” is no simple task.

How we contend with these issues will matter, because AI systems could have serious unintended impacts. This year, one paper in particular concerned people: ‘Automated Inference on Criminality Using Face Images.’ The researchers Xiaolin Wu and Xi Zhang claimed that machine learning techniques can be used to predict whether someone is a convicted criminal based on nothing more than a headshot — with 90% accuracy.
The authors, who sought to defend their methods, said that any resemblance to the physiognomy of the 19th century is purely accidental, and that “like most technologies, machine learning is neutral.” That’s a claim that provokes much skepticism, and has been roundly critiqued for years by many academics.
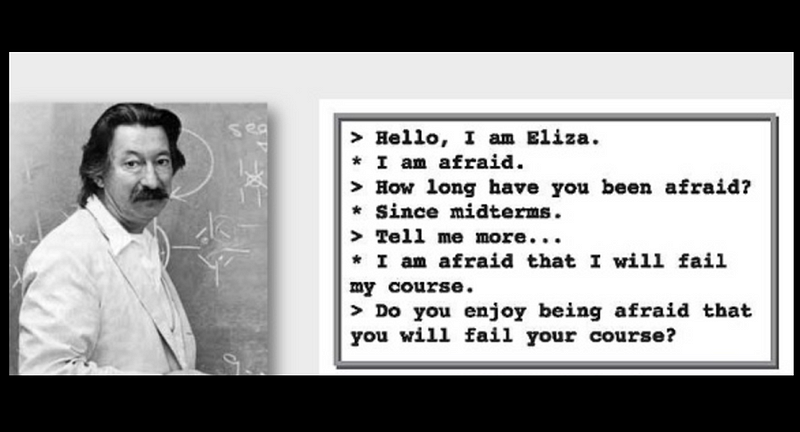
Even the early pioneers of AI were concerned about the myth of neutrality and objectivity. Joseph Weizenbaum was the man who invented ELIZA — the first conversational chat-bot — back in 1964 at MIT. It was a total hit. But its success also deeply concerned him: in particular, the “powerful delusional thinking” that artificial intelligence could induce — in both experts and the general public.
We can see this phenomenon at work in “automation bias”: the tendency for humans to accept decisions from automated systems, on the assumption that they are more objective or accurate, even when they are shown to be wrong. It’s been seen in intensive care units, nuclear power plants, and now we should ask if it might influence the justice system through algorithmic scoring.
An example of automation bias in action was shown in a RAND study on predictive policing in Chicago. After studying the system for a year, they found that it was completely ineffective at reducing violent crime. But it did have one significant impact: it increased the harassment and arrest of people on the ‘heat list.’ And, just as Joseph Weizenbaum feared, police incorporated it, even as it was failing them.
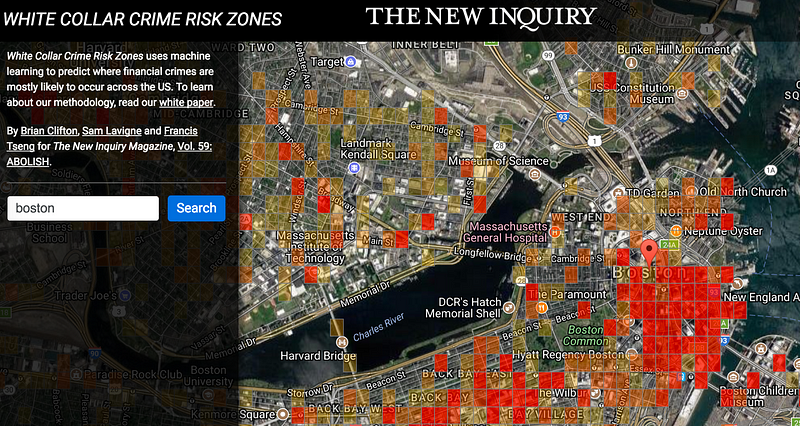
On the topic of predictive policing and bias, one of our favorite interventions of the year was this — the White Collar Crime app. It reverses who is typically visible and invisible in predictive policing data, by focusing just on the rich and powerful. It maps financial crime data from FINRA against every neighborhood in the US. Suddenly, Boston’s downtown financial district is exposed as a hotbed of crime!

Also this year, the AI field slowly began to confront its own issues of bias. New efforts such as AI4ALL are directly tackling problems of diversity and inclusion. Co-founded by AI Researchers Fei-Fei Li and Olga Russakovsky, AI4ALL partners with top universities to give young women and people of color the opportunity to understand and design their own AI systems.
So while there’s a long way to go, there are signs of progress — which our first panel of the evening will discuss.
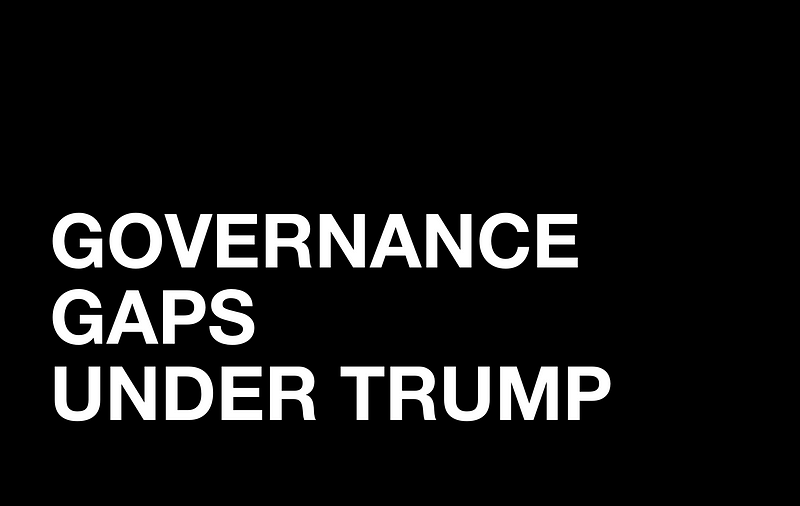
We now turn to our second topic — Governance Gaps Under Trump. And it requires a moment of real talk from us.
This time last year, we chaired the first AI Now Symposium in partnership with the Obama White House’s Office of Science and Technology Policy. This was part of a wider Administration approach to develop cutting edge policy around AI.
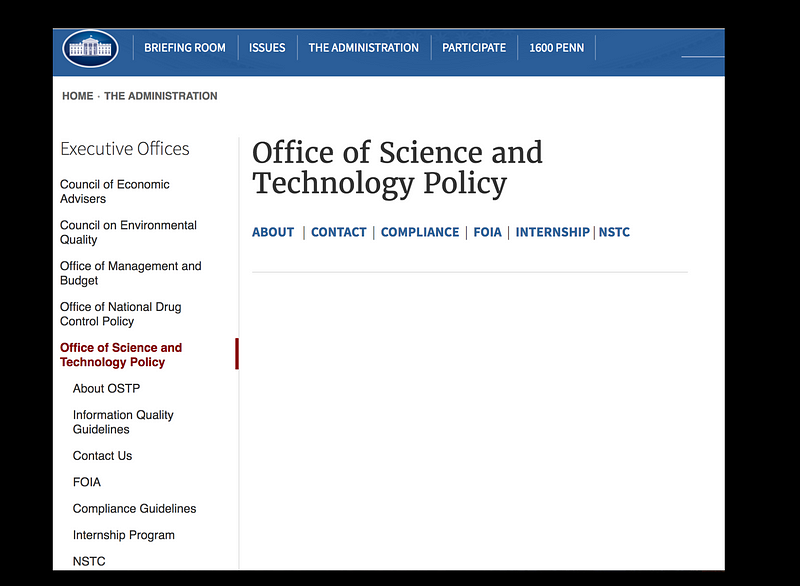
That process has stalled. OSTP is no longer actively engaged in AI policy, nor much of anything, if you believe their web site. And other parts of the administration aren’t picking up the slack. The current Treasury Secretary has said that the effect of AI on labor is not even on his radar.
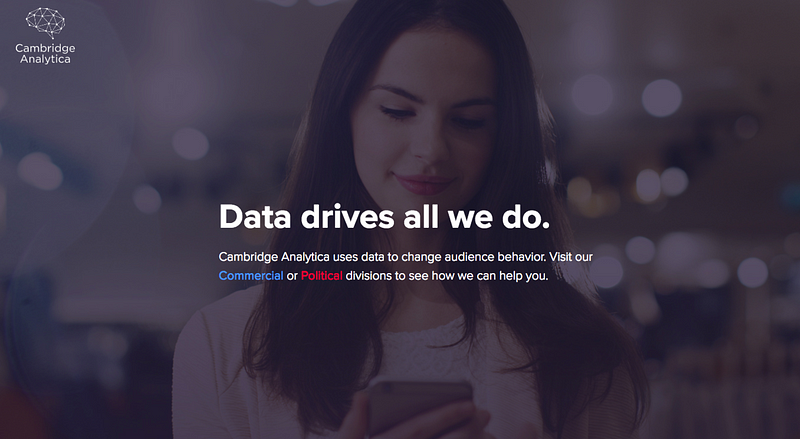
But this lack of a reality-based policy agenda for AI doesn’t mean that AI isn’t impacting politics. Cambridge Analytica, a controversial data firm that offers to manipulate “audience behavior”, claims to have massive individual profiles on 220 million people living in America —so, basically everyone. And depending on who you believe, they may have played a role in both the Trump and Brexit wins. But even if you don’t think that Cambridge Analytica is effective at manipulating voter intentions, there are real reasons for concern. And now, calls for accountability are now coming from inside the house.
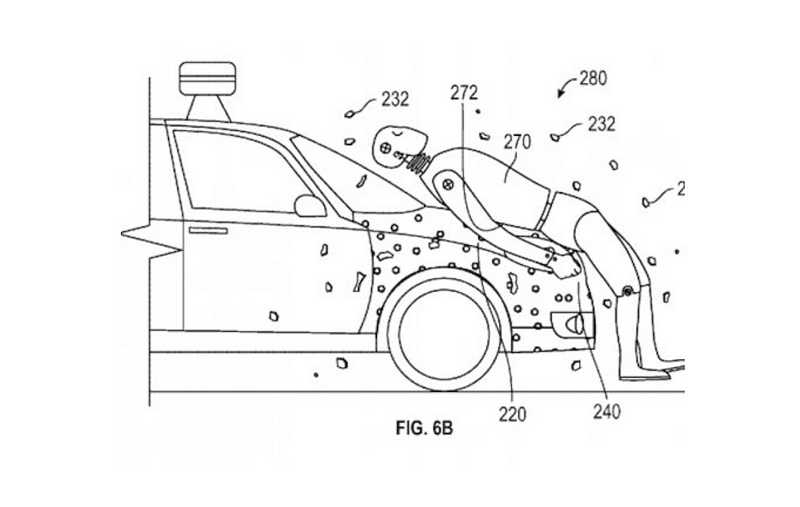
In the keynote at this year’s ACM Turing Awards —imagine The Oscars, but for computer science — Ben Shneiderman called for a National Algorithm Safety Board, which would monitor and assess the safety of algorithmic systems are they are applied to our social systems.
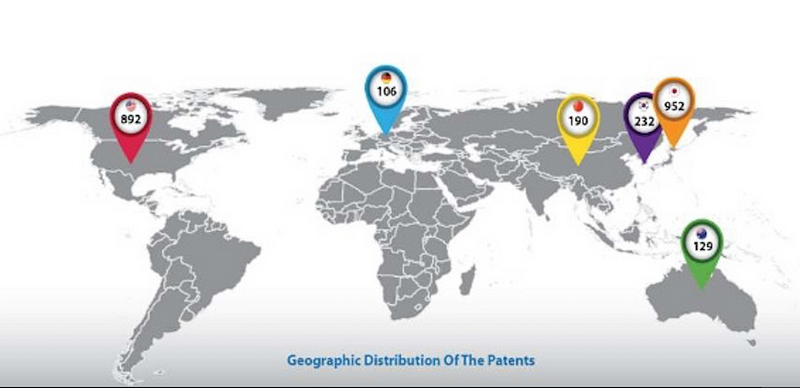
And these impacts will be complex. At a time of rising wealth inequality, a new tension is emerging in geopolitical power: the global north are rapidly becoming the AI haves, and the global south are becoming the AI have nots. This deepening of global power asymmetries will present complex international governance challenges in the next decade.
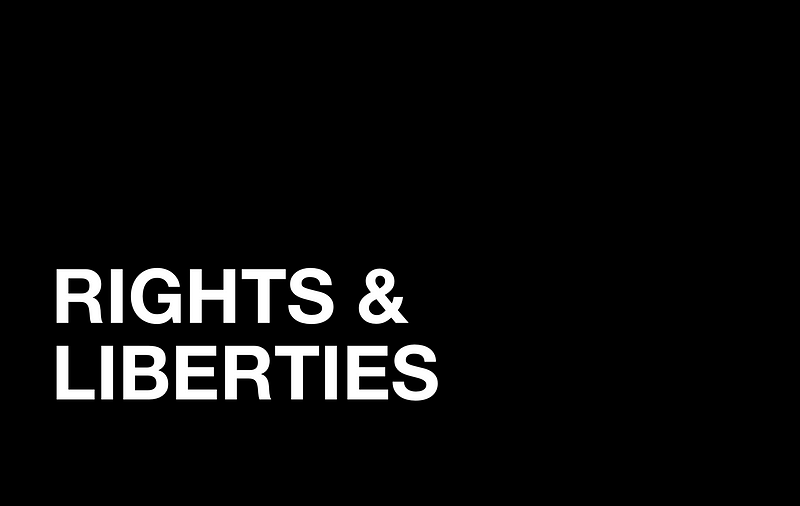
Now we turn to the last topic of the night, Rights and Liberties, which the final panel will address.
To set the scene, cast your mind back to June 2017, when Dubai’s first robocop reported for duty, complete with facial recognition systems to ID everyone it sees. This will be the first of many. If all goes according to plan, 25% of the Dubai police force will be robots by 2030.

And US law enforcement isn’t far behind. We’re already seeing a marked acceleration in the use of computer vision, mobile sensors, and machine learning.
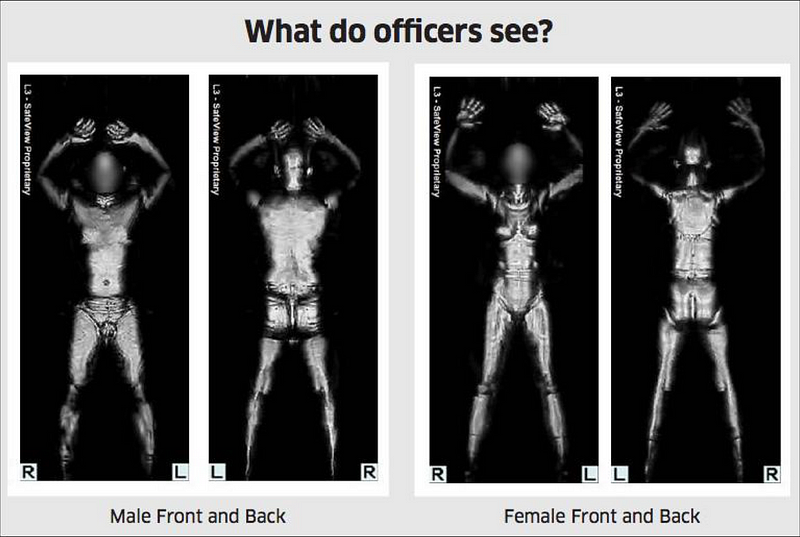
The Department of Homeland Security is hosting a Kaggle competition offering prize money to anyone who can make an algorithm to improve TSA’s detection of threats hidden under “a variety of object types, clothing types, and body types.” At the same time, the Director of National Intelligence, who oversees the nation’s spy agencies, is hosting its own contest, looking for “the most accurate unconstrained face recognition algorithm.”

But this isn’t all contests and aspirations. These systems are being built into the core of government services. Palantir, the data analytics firm co-founded by venture capitalist and Trump advisor Peter Thiel, is building a machine learning platform for ICE. It is scheduled to come online this September, and will allow ten thousand agents at a time to have access to incredibly sensitive information about millions of people’s addresses, places of work, social networks and biometrics. This could be a powerful engine for mass deportation in the US.
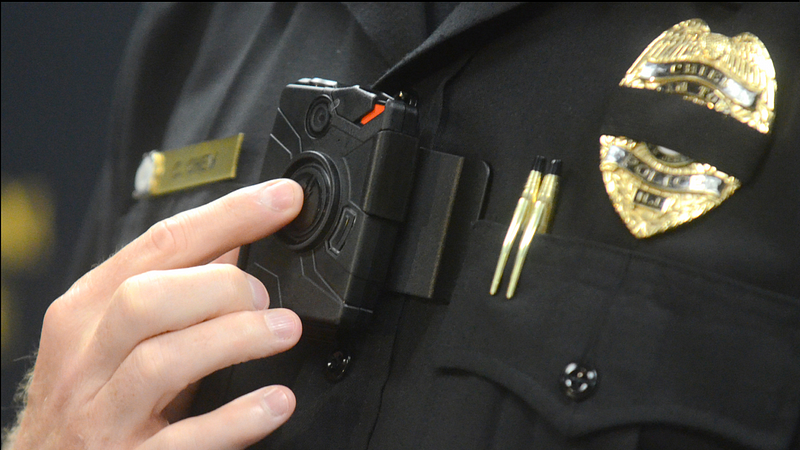
At the local level, Taser, the maker of stun weapons and police surveillance equipment, recently changed its name to Axon and rebranded as an AI company. It’s now working to add real-time facial recognition to police body cams, identifying anyone who encounters a police officer. They even claim to be able to “anticipate crime before it happens.”
Meanwhile, more than half of US adults already have their image stored in a law enforcement database, most of whom have never committed a crime. What rights to due process will people have when facing a system that can pull up their record before they’ve been considered a suspect?
But here are more signs of progress: the judicial system is beginning to recognize the importance of accountability.
This May, a judge in Texas ruled that teachers have a due process right to challenge the results of algorithmic performance rankings. This is a big case to watch, especially as it intersects with ongoing research and policy questions about AI explainability and bias.
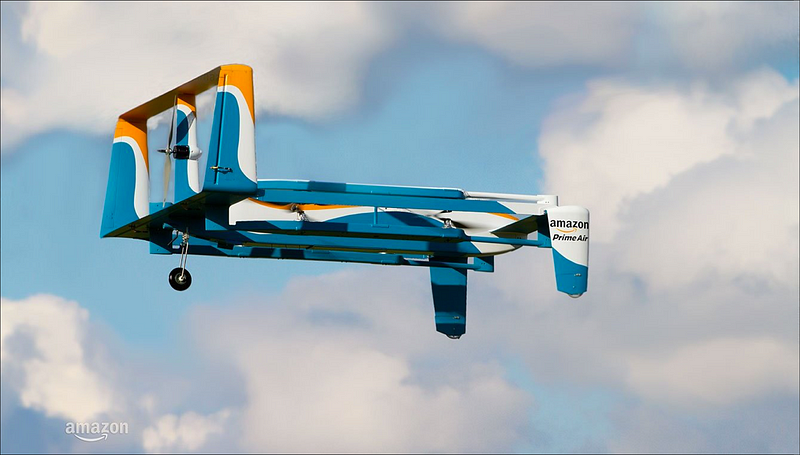
Shifts in labor practices and increasing automation also present significant challenges for rights and liberties. We’re all familiar with stories about robots replacing human workers. Like the one about Amazon working to “disrupt” global logistics by automating everything from forklifts to delivery workers, to trucking, one of the most common jobs in the US.
It’s notable that this comes at exactly the same time as low-wage workers are organizing for higher wages and better working conditions in campaigns like Fight for 15. This raises hard questions about the future of labor rights.
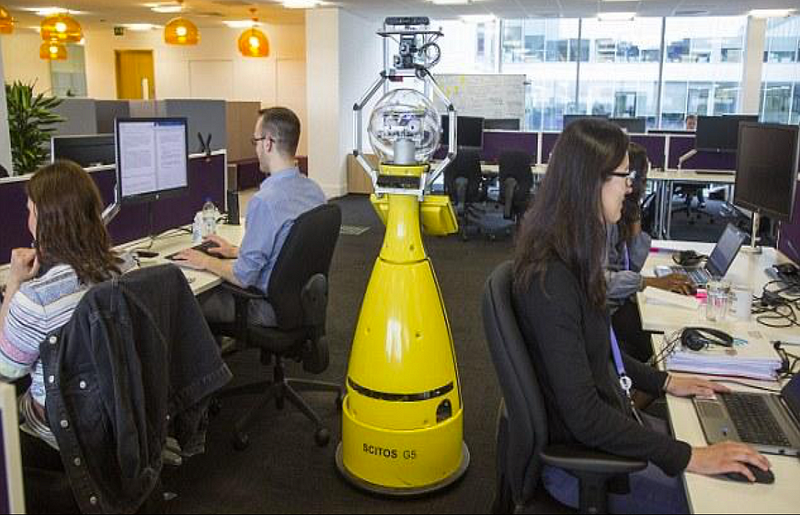
But the story of AI and labor is much more complicated than “find and replace” humans with robots.
AI is augmenting workers and workplaces, creating human-AI collaborations. AI systems are also judging workers, recommending who to hire and who to fire. At this year’s We Robot conference, Garry Mathiason, an Employment and Labor Partner at global employment law firm Littler Mendelson P.C., shared research predicting that within five years, a full 80% of US companies will be using AI for recruiting and hiring. How will those systems be vetted for issues of bias or error?
In addition, AI systems are playing a role in shifting the way we work, often without us even noticing.
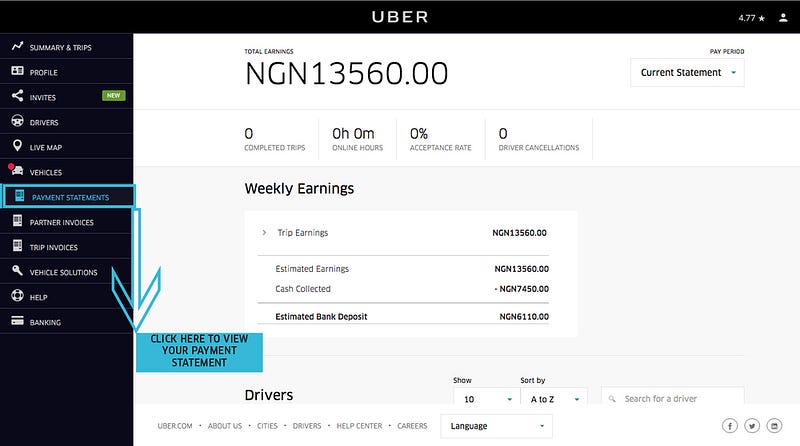
This is what was happening at Uber, which has been using its vast data troves and applying behavioral economic models to “nudge” drivers into working longer hours. This is another example of how much power a centralized platform can have when it can see and control worker data down to the individual level.
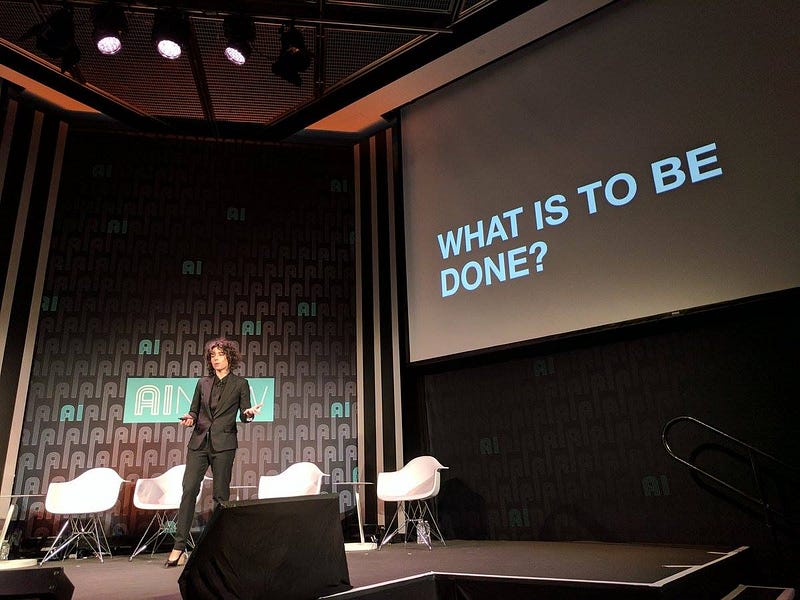
So we just presented you with a rapid series of research studies, investigative findings, and industrial decisions. And, remember, all these stories come from just the last year.
So what is to be done? AI is weaving its way through everyday life, and yet we know little about its full impacts and implications. And before we can know, we need to measure. This is not the kind of measurement where you simply instrument a server to collect a new variable. This will require drawing on diverse methods from across disciplines to create shared understandings of AI’s powerful implications.
Which leads us to our announcement for the night.
We are launching the AI Now Initiative, a new research institute based in New York. It will conduct empirical research on artificial intelligence across four key areas: bias and inclusion, labor change and automation, critical infrastructure and safety, and basic rights and liberties.
We will be inviting academics, lawyers, AI developers, and advocates to join us in addressing these issues. And we’re delighted that our first partner is the ACLU, who are committed to understanding the effects of advanced computation on civil liberties.
So watch this space, and if you are interested in working on these topics too, come and say hello. It’s this community who are doing the essential research, activism and technical design that will help to address these issues. Together we can build the field that will work to understand the complex social implications of AI and ultimately build better AI systems.
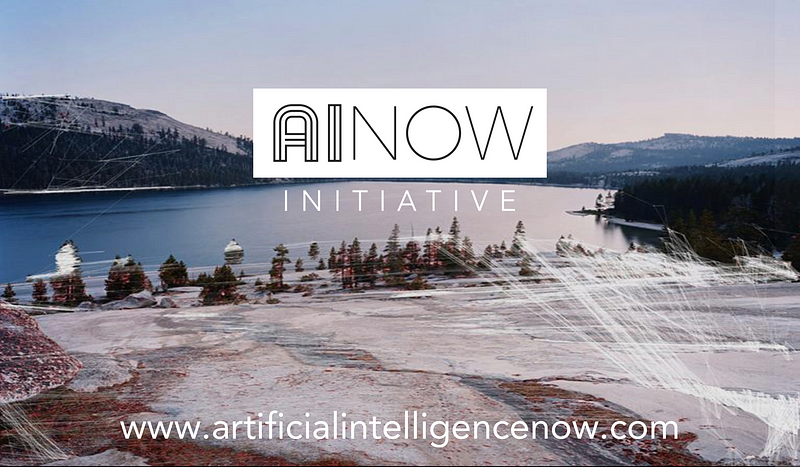