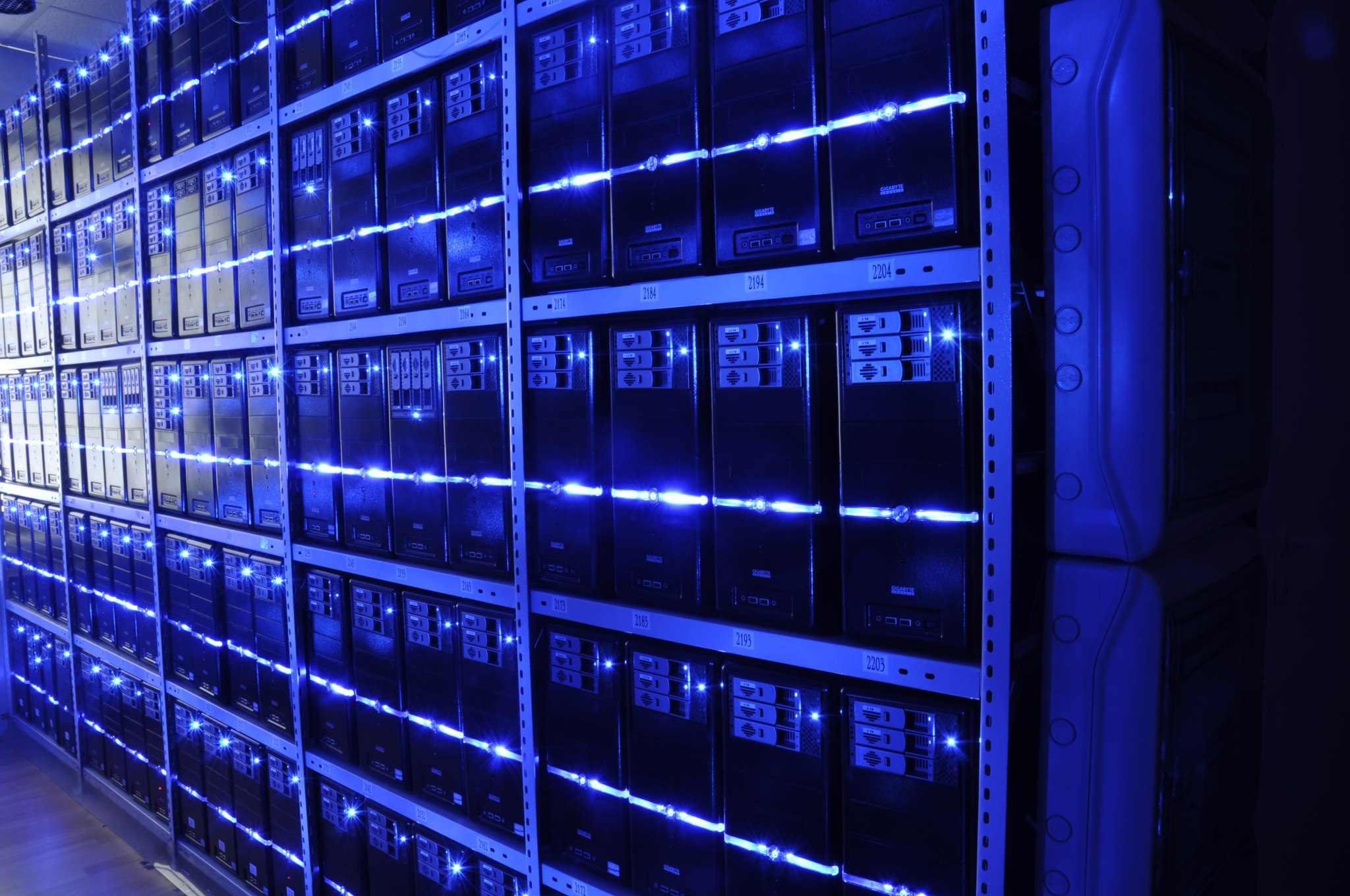
Suspect Development Systems: Databasing Marginality & Enforcing Discipline
Jul 7, 2021
Databasing Marginality & Enforcing Discipline
By Rashida Richardson & Amba Kak
This piece provides a summary overview of our new article “Suspect Development Systems: Databasing Marginality and Enforcing Discipline,” available on SSRN here, and forthcoming in the University of Michigan Journal of Law Reform. This article received the Reidenberg-Kerr Award for the best paper by pre-tenured scholars, at the Privacy Law Scholars Conference, 2021.
What connects gang databases in the UK and US, the recent Argentinian police database of minors, or the decades long practice of “habitual offender” paper files maintained in police stations in India with the UK’s recent Universal Credit system for welfare delivery or citizenship and ID databases across the world? Amid the heightened public concern, advocacy campaigns, and institutional funding afforded to systems described as artificial intelligence (AI) or as algorithmic/automated decision-making systems (ADS), database infrastructures and the classificatory logics that underpin them are often relegated to the margins.
Each of these government database projects encodes key determinations about who is included and who is excluded from the vision of the nation state. These infrastructures facilitate profiling, stigmatizing, or even eliminating those who are cast as “suspect”. Similar to the creation and maintenance of suspect categories of people — like the habitual offender or the gang member in the criminal justice system — we see racialized tropes like “the welfare dependent mother” or “the doubtful citizen” replicated from one database to the next, in ways that ensure these stigmatizing labels travel through multiple realms of governance and the private sector as well. In criminal justice databases, inclusion in the database can result in criminalization and exclusion; whereas in welfare or citizenship databases, it is exclusion or being removed from databases that can serve to punish, criminalize, or otherwise disempower people and groups. The bureaucratic form of these systems of classification can also conceal the punitive nature of the forms of control that they enable, masking the looming and omnipresent threats of scrutiny, harassment, and violence that often accompanies being included, or excluded, from these systems.
Yet in popular and policy discourse databases are characterized as the foundational and passive raw material that enable the creation of more advanced algorithmic systems that can sort, prioritize, predict and so on. Despite the fact that the terms AI and ADS are notoriously vague and hard to define, databases are still invariably positioned in the dominant scholarship as in a necessary but “subordinate position” to these more recent systems. These vague technological thresholds around what does or does not “count” as AI have policy implications as well, given heightened regulatory scrutiny of these systems in domains like policing, education, and employment. To illustrate, even as the Chicago Police Department announced it will no longer use its new and controversial “predictive policing” program in response to public criticism, it proudly announced plans to revamp its much-criticized gang database, which serves a similar role insofar as these databases also identifying people or groups as in need of heightened scrutiny and policing based on the risk they are predicted to pose “in the future.”
These vague technological thresholds around what does or does not “count” as AI have policy implications as well, given heightened regulatory scrutiny of these systems in domains like policing, education, and employment. To illustrate, even as the Chicago Police Department announced it will no longer use its new and controversial “predictive policing” program in response to public criticism, it proudly announced plans to revamp its much-criticized gang database, which serves a similar role insofar as these databases also identifying people or groups as in need of heightened scrutiny and policing based on the risk they are predicted to pose “in the future.”
Creating a common discourse: SDS as a categorical term and lens
Responding to this gap in the discourse, we introduce the term “Suspect Development Systems” (“SDS” for short) as a new normative category to describe these database systems. SDS can be defined as (1) information technologies used by government and private actors; (2) constructed to manage vague and often immeasurable social risks based on presumed or real social conditions (e.g. violence, poverty, corruption, substance abuse); (3) and which subject targeted individuals or groups to greater suspicion, differential treatment, and more punitive and exclusionary outcomes.
This proposal for recasting these systems as SDS is, we believe, a valuable intervention for many reasons:
- It works to de-center technical thresholds and bridge temporal and geographical divides: SDS can create a common discourse around technological systems which does not rely for its validity on specific technological capabilities, and thus bridges geographical and temporal divides. The term also allows us to draw continuities between historical practices, whether it is linking current gang database practices in the U.K. to the British colonial strategy of criminalizing entire communities (designated “criminal tribes”) in India, or lessons from the use of watchlists to suppress political dissent in the United States during Civil Rights and anti-Vietnam war protests.
- It names things plainly: SDS is a deliberately normative category. Unlike descriptors or incomplete technical terminology commonly used for these systems (i.e. databases, AI or ADS), SDS calls attention primarily to how these technologies and practices amplify structural inequities and the modes through which they discipline and control individuals and groups (i.e. suspect development) The particular means by which they accomplish this is secondary.
- It productively links criminal/carceral systems and other spheres of governance: Governments across the world are turning to punitive measures to respond to a range of socio-economic problems like social welfare, education, or immigration, which often encourage practices of criminalization and exclusion in domains well outside of criminal justice. As “technologies, discourses, and metaphors of crime and criminal justice” have crept into multiple spheres of governance, SDS as a category can be wielded strategically across domains.
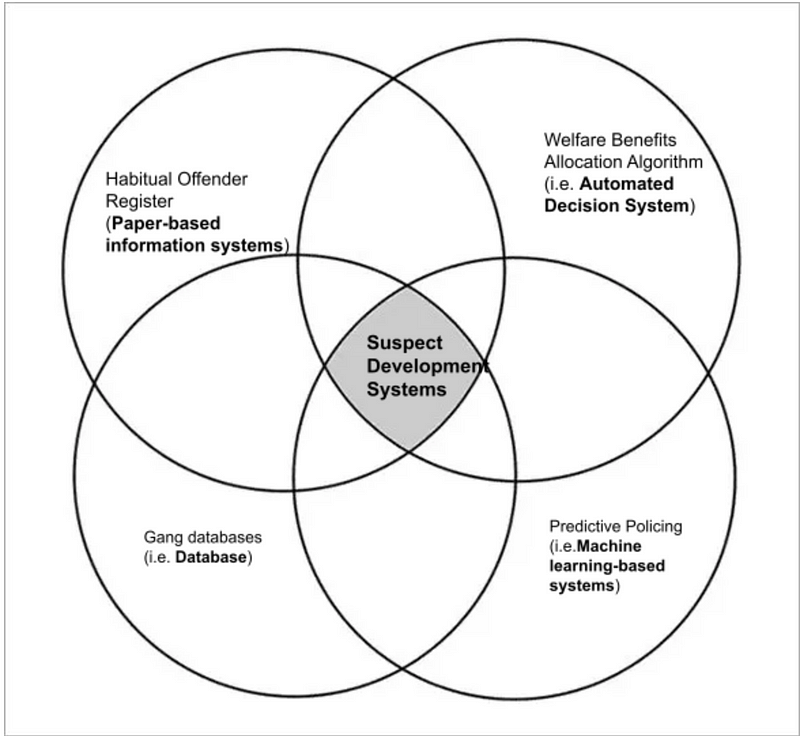
Prompting a deeper analysis of harm: Suspect Development Systems as a framework for analysis (in the criminal justice system and beyond)
SDS is more than a category with which to identify and name these systems. In our Article, we outline a systematic framework with which to understand and evaluate them. We drew inspiration from gaps in how algorithmic systems are analyzed, especially in the burgeoning policy discourse around “algorithmic accountability”.
SDS is more than a category with which to identify and name these systems. In our Article, we outline a systematic framework with which to understand and evaluate them. We drew inspiration from gaps in how algorithmic systems are analyzed, especially in the burgeoning policy discourse around “algorithmic accountability”. The recent and growing emphasis on a deeper and contextual understanding of the social impacts of algorithmic systems is welcome, however, emergent policy frameworks like Algorithmic Impact Assessments (AIAs) and algorithmic audit are still limited in their scope of review and analysis. These mechanisms struggle to effectively surface the structural inequities and tacit modes of discipline, control, and punishment that can be amplified and enacted through such systems.
The SDS framework expands the contours of analysis by including five feature categories: technical, legal, political economy, organizational, and social [See below for a table summary]. These categories were developed through close examination of criminal intelligence databases used by law enforcement and other government actors to profile and target individuals and groups considered to be a social risk for criminality in the United States, United Kingdom, and India. This framework can both inform civil society advocacy and research agendas, and be operationalized by state and private actors tasked with deciding whether (and how) to develop and use such systems, or with governing relevant spheres of influence.
While we developed the SDS framework using paradigmatic examples from the criminal justice system, we argue that applying it to systems of social welfare, citizenship, and universal databases offers both tactical benefits and helps us arrive at a more nuanced understanding of how these systems operate, as we see here:
- Welfare distribution: Despite the origins of welfare distribution as a socially inclusive area of governance, the use of databases in this sphere has coincided with a global shift to a welfare state characterized by assumed scarcity, and increasingly focused on eliminating “risky” people and behaviors, along with requisite taxonomies of risk and exclusion. In this context, welfare databases qualify as SDS.
- National biometric ID systems: are currently being promoted across the Global South under an inclusive development mandate. These have had disproportionately exclusionary outcomes and work to legitimize differential treatment of people that are already subject to intersecting forms of marginality. Here, the act of naming these systems as SDS can offer tactical benefits to the already heated civil society resistance to these projects, helping stakeholders understand their normative function.
- SDS in immigration control: The fact that immigration policies and practices are increasingly focused on securitization and criminalization is well-studied as a global phenomenon. We use the SDS framework to explore how recent citizenship database projects facilitate broad-based suspicion, exclusionary outcomes, and new forms of precarity for those that are deemed suspect. Our analysis in this section also demonstrates how the SDS framework can be used to assess risks and concerns associated with technological systems still under development.
CONCLUSION: Suspect Development Systems as a tactical intervention
We encourage technology developers, policymakers, legal practitioners, government officials, scholars, advocates, and community members to apply SDS as a term and as an analytical framework. We believe that it can help inform and actively change the trajectory of SDS design, use, and outcomes. An SDS-based analysis can also aid assessments of proposed technological solutions to complex social policy concerns, specific technology policy proposals, in addition to helping identify alternative opportunities or locations for interventions.
While SDS use is global and our cross-jurisdictional analysis demonstrates common challenges, there is of course no universal panacea. However, in light of the growing global discourse regarding banning or significantly limiting the use of some AI technologies that are demonstrably harmful (e.g. autonomous weapon systems or facial recognition technologies), the SDS framework can aid these conversations, working to establish international norms that can inform domestic policies.
Table 1 — SDS Taxonomy
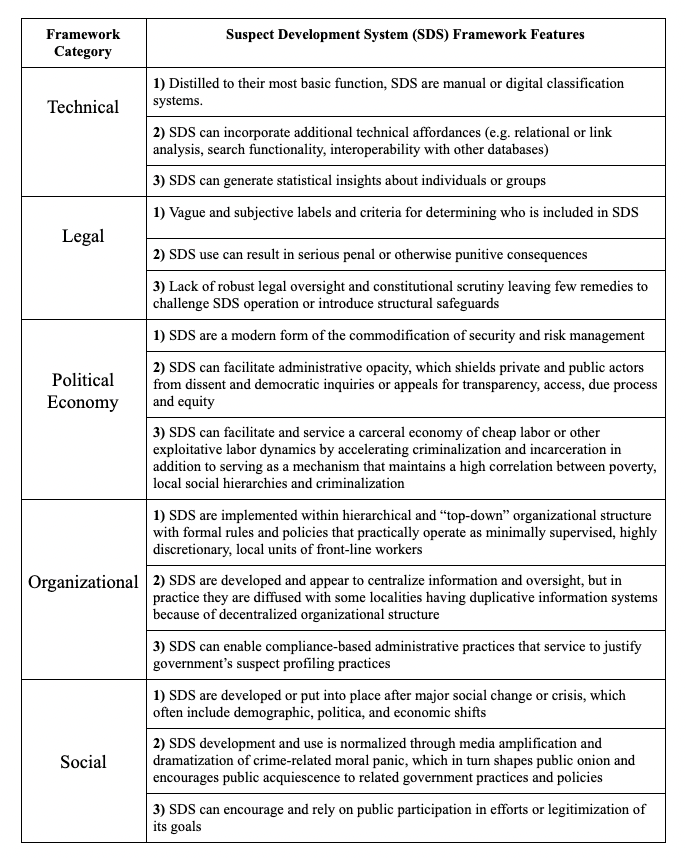
Amba Kak is Director of Global Policy & Programs at the AI Now Institute. She can be found at @ambaonadventure.
Rashida Richardson is Assistant Professor of Law and Political Science at Northeastern University. More on her here.