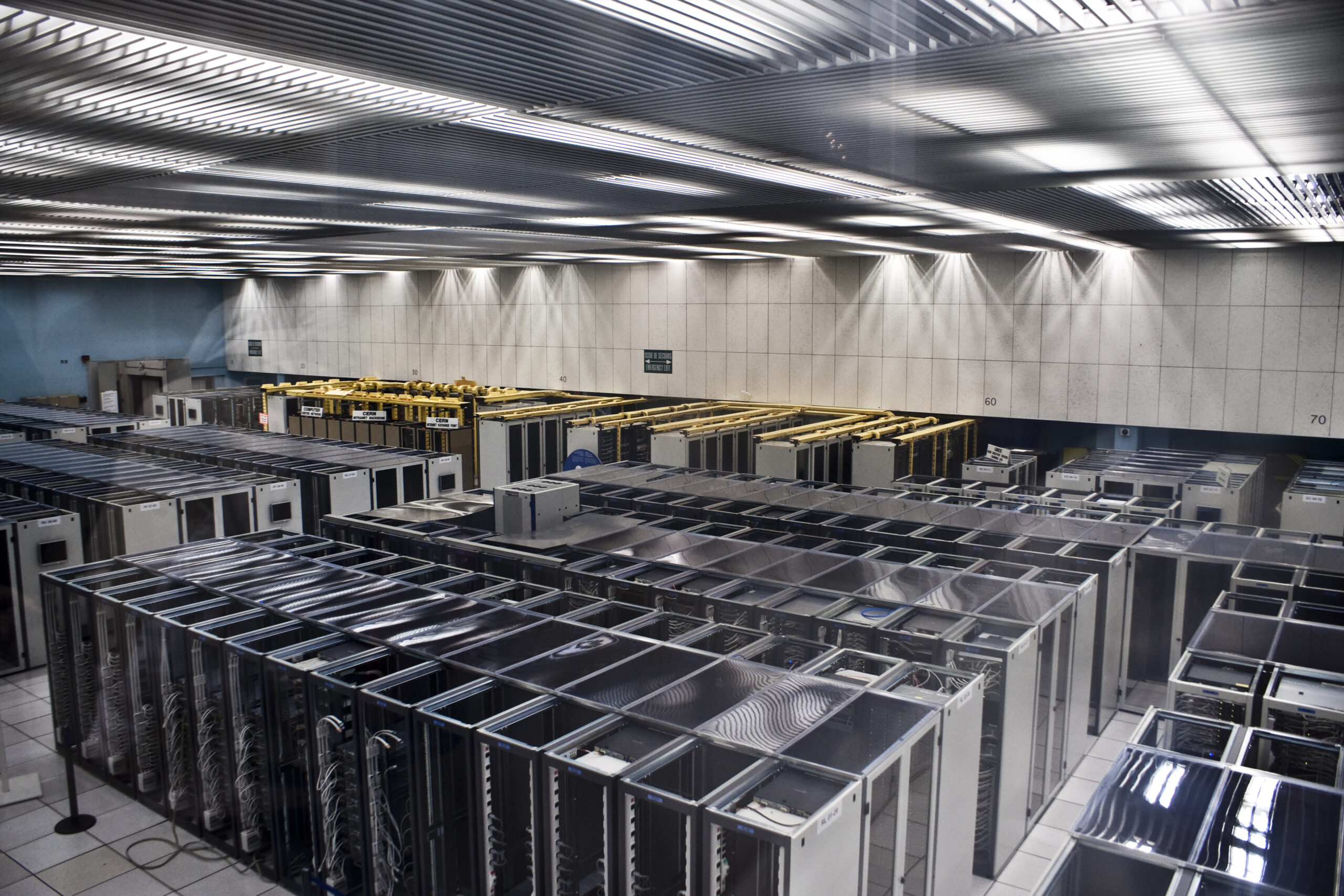
AI Now Managing Director Sarah Myers West Gives Remarks Before Heads of Agency, International Competition Network
Oct 18, 2023
On October 18th, 2023, AI Now’s Managing Director Sarah Myers West gave remarks to the International Competition Network. The full statement is below.
Thank you for having me here today, it’s an honor to be addressing this group. I was asked to provide opening remarks that help to frame how to understand artificial intelligence, and the implications of AI for competition. If there is one point I’d like to make today, it is that in the midst of a tremendous amount of regulatory attention to AI, from a number of different policy domains – from data protection and privacy, to labor, to copyright, to consumer protection – there is no issue more important than effectively tackling the significant concentration of power in AI and its effects on the market, incentivizing a race to the bottom at great cost to the public.
I’d like to start by taking a step back: what is artificial intelligence in the first place? This term is not one that should be taken for granted: it is imbued with significant weight, as though it evolves of its own accord. But as the FTC has rightly pointed out, AI is best understood as a marketing term. It has come to mean different things over the course of an almost 70-year history, from expert systems to robotics to neural networks and now large-scale AI. This lack of a fixed understanding of artificial intelligence has been misused by firms in this moment of considerable AI hype; the FTC among others has taken action against firms that mislead consumers by improperly describing their products as being built using AI.
In its current form, AI is best understood as a set of computational processes that use statistical techniques in combination with very large datasets, powered by the engine of ever growing amounts of computational power. As the writer Ted Chiang recently put it, AI may be best described as ‘applied statistics’.
This is true even of the generative AI systems that have captured everyone’s imaginations over the last several months, feeding the frenzy of anxiety and excitement about AI. What’s notable about generative AI – systems like ChatGPT and Bard – is that it provides an interface through which consumers and enterprise users interact with AI, using it to produce new material whether text or images or video. While the underlying techniques are more of a continuation than a radical break with prior forms of AI, this has transformed AI from something that’s invisibly used on us – through facial recognition or automated decisionmaking – to something that we can directly use.
Having this material definition of AI is important because it enables us to understand the present moment as an evolution of an existing market, rather than the emergence of a novel one. Today’s AI boom is driven at its core by commercial surveillance, and its incentive structures are shaped by the existing infrastructural dominance by a small handful of firms. This is what is driving the push to build AI at larger and larger scale, increasing the demand for resources that only Big Tech firms can provide and further cementing their considerable advantage.
It’s worth then unpacking the current landscape surrounding competition in AI with that stack in mind: there are heavy levels of concentration at every layer, from the firms that make chips (design firms like Nvidia and fabricators like TSMC) to those that run cloud infrastructures (Amazon, Microsoft, and Google, in focus for several of the agencies in the room), those who amass large datasets through sprawling ecosystems (Google, Amazon, Meta) and train base AI models (Google, Amazon, Meta, Microsoft – some of these through partnerships with firms like OpenAI or Anthropic).
There are some concerning trends that emerge from this picture of the market:
- First, the reliance on vertical integration across the AI stack. Big Tech firms that already have a data and cloud advantage are attempting to build out vertically integrated AI enterprises using exclusive agreements and strategic partnerships, like OpenAI’s deal with Microsoft which ensures its products run exclusively on Azure and Amazon and Google’s recent deals with Anthropic. Nvidia, in turn, is making its own vertical integration moves, using its dominant position in chip design to muscle its way in to the cloud computing market and steadily buying up AI firms.
- Second, the bundling together of hardware and software presents particular challenges. For example, one of the sources of Nvidia’s dominance in chip design is because the software they created to compile code onto their chips, CUDA, acts as a moat. In turn, tech companies are stretching deeper into hardware, trying to design their own chips that work seamlessly with their AI systems.
- Third, the emergence of the platform or marketplace model for AI model provisions is an area of concern given the sprawling ecosystems many AI firms operate. For example, Amazon runs its Bedrock marketplace, on which all of its product offerings operate using Amazon Web Services. On Bedrock it sells access to its own models, those of Anthropic, in which it recently took a large stake, and a handful of other companies’ models – as in other marketplaces this raises self-preferencing concerns.
Why does this matter?
For one, the market is already entrenched in favor of incumbents, which means a less accountable, and innovative AI ecosystem – with the potential to have grave impacts on the information environment, creative industries, and labor markets, among others. As it stands, ‘innovation’ can really only take place within this market at the edges, through ‘fine tuning’ of models built by entities with the resources – the big firms – over their APIs or ‘open source’ models, essentially giving them free product development – many folks in the room have ready grappled with how companies in the industry have utilized open source software to deepen rather than prevent competition, happy to dig deeper into that in the discussion.
This also raises concerns about single points of failure: having a small number of firms with outsized power in the AI market raises significant resilience and national security concerns. Flaws in one of the originating models could diffuse widely throughout the economy and impact any number of the systems built on top.
In our recent report on computational power and AI we outline a broad set of recommendations for addressing these concerns. But I’d like to highlight a few in particular for consideration here:
- First, in order to effectively tackle AI concentration, we need to disrupt the silos between policy domains. For example, data policy is a particularly important lever for creating limitations on firms’ market dominance. Interventions such as limiting first party data collection are critical to curbing the continued expansion of AI at larger and larger scale, and particularly targeting the linkages between consumer surveillance and competition harms. Relatedly, prohibitions on the secondary use of data collected from users for the sole purpose of training AI models would get at a widespread practice that undermines the conditions under which consumers consented to using a technology in the first place. Labor policy also offers important inroads, such as the prohibition of non-competes in order to prevent talent bottlenecks in AI, given the specialized knowledge required to train these systems.
- Second, ex ante approaches are the need of the moment for competition and other AI harms, from strengthening merger review of AI acquisitions by Big Tech firms to pre-market scrutiny of AI systems before they are publicly released. Many of those in the room have seen and experienced how challenging it is to roll back anti-competitive behavior by tech firms long after it has taken place. Wait and see approaches risk allowing incumbent players to entrench their dominance even more fully, alongside other related social and economic harms. Rather than attempt to roll this back years from now, there is a prime window now for intervention.
- Structural interventions are the right path forward for this market. It is not a consumer-facing market: given the high cost of credit, companies are targeting enterprise users predominantly and vertical integration and ecosystem ownership as their core strategies. The most effective means of tackling these dynamics will be through structural methods.
A final point I’d like to leave you with today: there is nothing about the current trajectory of AI that we should regard as inevitable. While the current AI market is structurally inclined towards concentration of power in a handful of companies, it is important to remember that this is not the only way to build AI – there is nothing inevitable about the way technologies are being developed, and we should treat claims to this effect with skepticism. There’s tremendous scope for regulatory friction to redirect the current trajectory.