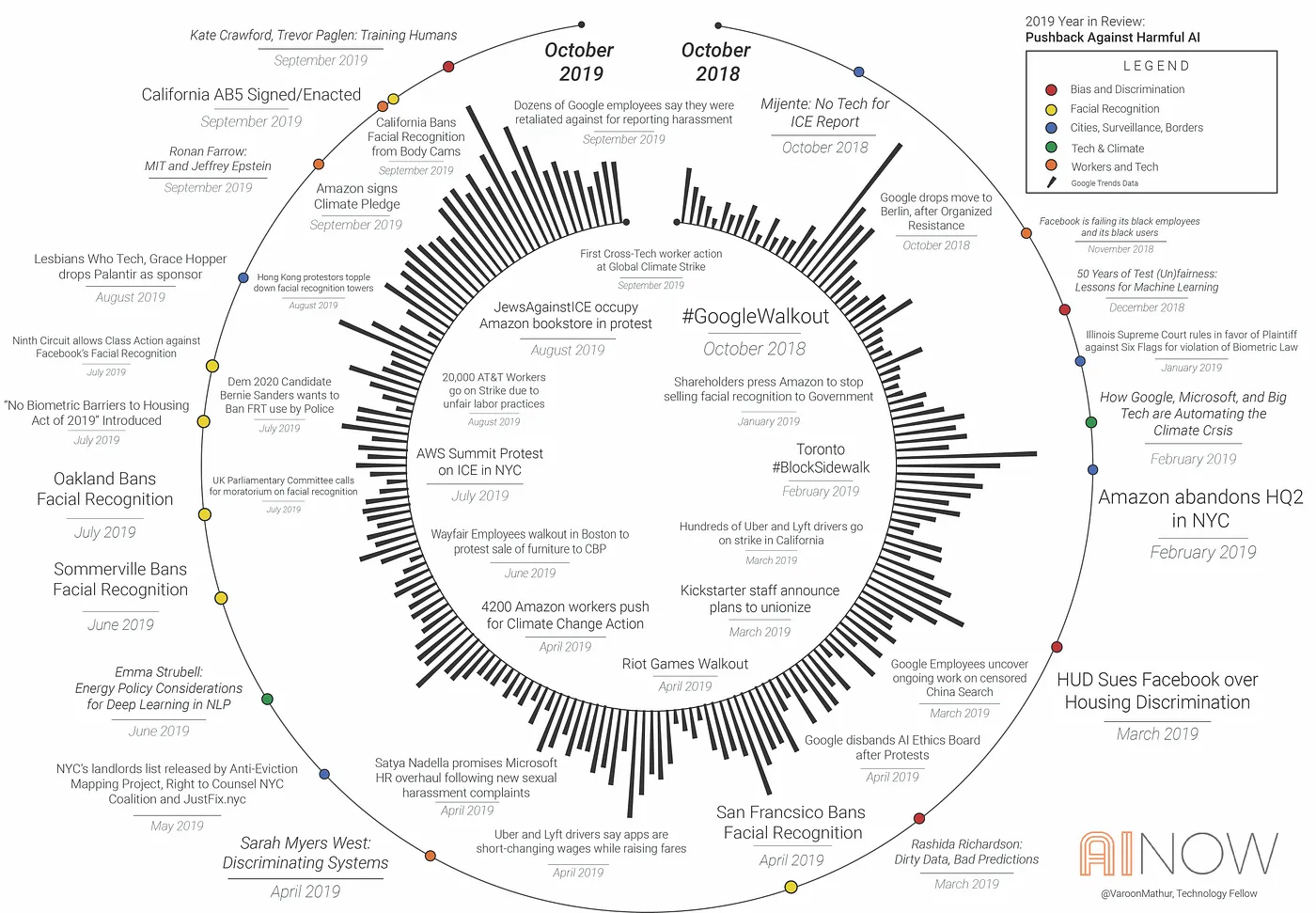
The Growing Pushback Against Harmful AI
On October 2nd, the AI Now Institute at NYU hosted its fourth annual AI Now Symposium to another packed house at NYU’s Skirball Theatre. The Symposium focused on the growing pushback to harmful forms of AI, inviting organizers, scholars, and lawyers onstage to discuss their work. The first panel examined AI’s use in policing and border control; the second spoke with tenant organizers from Brooklyn who are opposing their landlord’s use of facial recognition in their building; the third centered on the civil rights attorney suing the state of Michigan over its use of broken and biased algorithms, and the final panel focused on blue-collar tech workers, from Amazon warehouses to gig-economy drivers, to speak to their organizing and significant wins over the past year. You can watch the full event here.
AI Now co-founders Kate Crawford and Meredith Whittaker opened the Symposium with a short talk summarizing some key moments of opposition over the year, focusing on five themes: (1) facial and affect recognition; (2) the movement from “AI bias” to justice; (3) cities, surveillance, borders; (4) labor, worker organizing, and AI, and; (5) AI’s climate impact. Below is an excerpt from their talk.
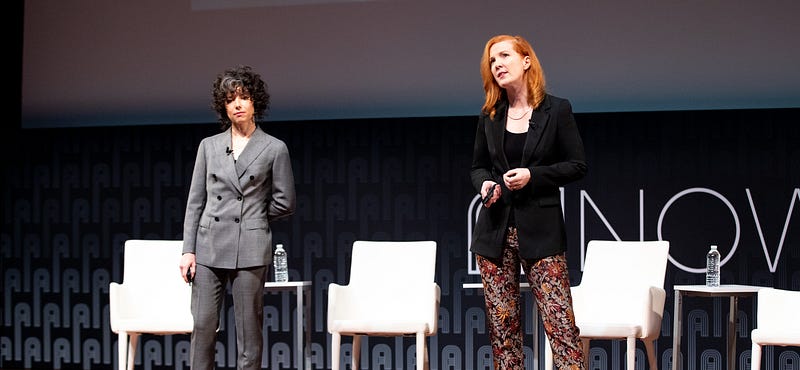
1. FACIAL AND AFFECT RECOGNITION
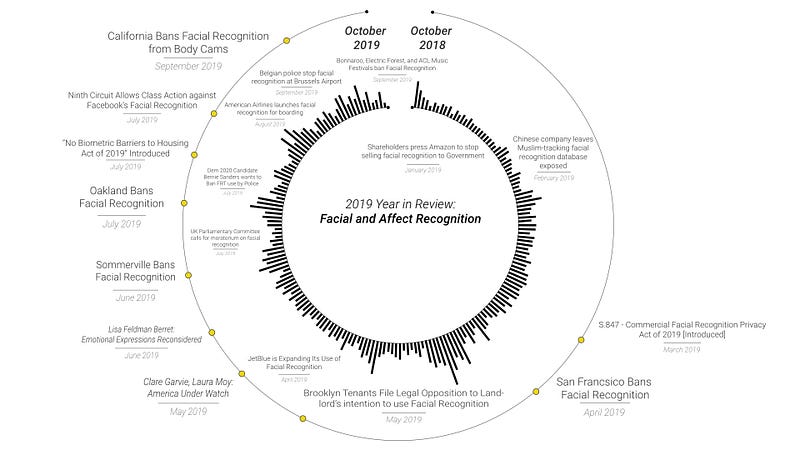
This year, in 2019, companies and governments ramped up the rollout of facial recognition — in public housing, in hiring, and in city streets. Some US airlines are now even using it instead of boarding passes, claiming it’s more convenient.
There has also been wider use of affect recognition, a subset of facial recognition, which claims to ‘read’ our inner emotions by interpreting the micro-expressions on our face. As psychologist Lisa Feldman Barret showed in an extensive survey paper, this type of AI phrenology has no reliable scientific foundation. But it’s already being used in classrooms and job interviews — often without people’s knowledge.
For example, documents obtained by the Georgetown Center on Privacy and Technology revealed that the FBI and ICE have been quietly accessing drivers license databases, conducting facial-recognition searches on millions of photos without the consent of individuals or authorization from state or federal lawmakers.
But this year, following calls to severely limit the use of facial recognition from scholars and organizers like Kade Crockford at the ACLU, Evan Selinger of Rochester Institute of Technology, and Woodrow Hertzog of Northeastern University, voters and legislators started doing something about it. The Ninth Circuit Court of Appeals recently ruled that Facebook could be sued for applying facial recognition to users’ photos without consent, calling it an invasion of privacy.
San Francisco signed the first facial recognition ban into law this May, thanks to a campaign led by groups like Media Justice. This was followed by two more cities. And now there’s a presidential candidate promising a nationwide ban, major musicians are demanding the end of facial recognition at music festivals, and there’s a federal bill called the No Biometric Barriers to Housing Act, targeting facial recognition in public housing.
Pushback is happening in Europe, too — a UK parliamentary committee called for trials of facial recognition to be stopped until a legal framework is established, and a test of these tools by police in Brussels was recently found to be unlawful.
Of course, these changes take a lot of work. We are honored to have organizers Tranae Moran and Fabian Rogers from the Ocean Hill Brownsville neighborhood with us tonight. They are leaders in their tenants association, and worked with Mona Patel of Brooklyn Legal Aid to oppose their landlord’s attempt to instal a facial recognition entry system. Their work is informing new federal legislation.
And let’s be clear, this is not a question of needing to perfect the technical side or ironing out bias. Even perfectly accurate facial recognition will produce disparate harms, given the racial and income-based disparities of who gets surveilled, tracked and arrested. As Kate Crawford recently wrote in Nature — debiasing these systems isn’t the point, they are “dangerous when they fail, and harmful when they work.”
2. FROM “AI BIAS” TO JUSTICE
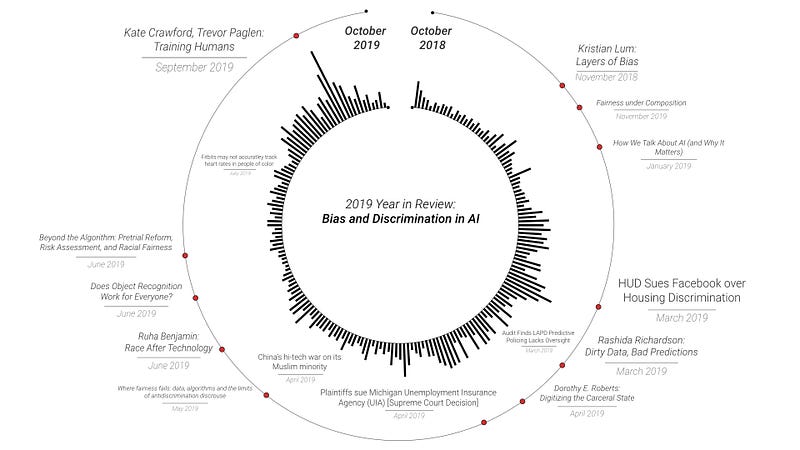
This year we also saw some important moves away from a narrow focus on technical-only ‘de-biasing’ of AI toward a more substantial focus on justice.
This has been driven in part by so many troubling examples.
For instance, former Governor Rick Snyder of Michigan, a tech executive and the person who presided over the Flint water crisis, decided to install an state-wide automated decision system called MiDAS. It was designed to automatically flag workers suspected of benefits fraud. Aiming to cut costs, the state installed MiDAS and fired its entire fraud detection division. But it turns out the MiDAS system was wrong 93% of the time. It falsely accused more than 40,000 residents, prompting many bankruptcies and even suicides. But MiDAS was just one part of a larger set of austerity policies focused on scapegoating the poor.
We’re honored to have Jennifer Lord with us tonight, the civil rights attorney who sued on behalf of the people whose lives were upended. She fought the MiDAS case all the way to the Michigan Supreme Court. At every step she faced political resistance, and tonight she will give us the full picture of why it was a more complex problem that just a faulty technical system. These issues are deeper than code and data.
Another example comes from research led by AI Now’s Director of Policy, Rashida Richardson, who examined the connection between the everyday work of police and predictive policing software. Her and her team found that in many police departments across the US, predictive policing systems likely used tainted records that came from racist and corrupt policing.
Clearly, correcting for bias in this case isn’t a matter of removing one or another variable in your dataset. It requires changing the police practices that make the data. This is something that researcher Kristian Lum of the Human Rights Data Analysis Group has also shown in her groundbreaking work on how algorithms amplify discrimination in policing. We’re delighted that she’s with us tonight.
On the same panel, we also have Ruha Benjamin of Princeton University. She recently published two extraordinary books, Race After Technology and the edited collection Captivating Technology. Alongside scholars like Dorothy Roberts and Alondra Nelson, she powerfully examines the politics of classification, how race is used to justify social hierarchies, and how these logics are feeding into AI.
Kate Crawford and AI Now Artist Fellow Trevor Paglen also interrogated the politics of classification recently in their Training Humans show, the first major art exhibition looking at the training data used to create machine learning systems. The show examines the history and logics of AI training sets, from the first experiments in 1963 by Woody Bledsoe through to the most well-known and widely used benchmark sets, like Labelled Faces in the Wild and ImageNet.
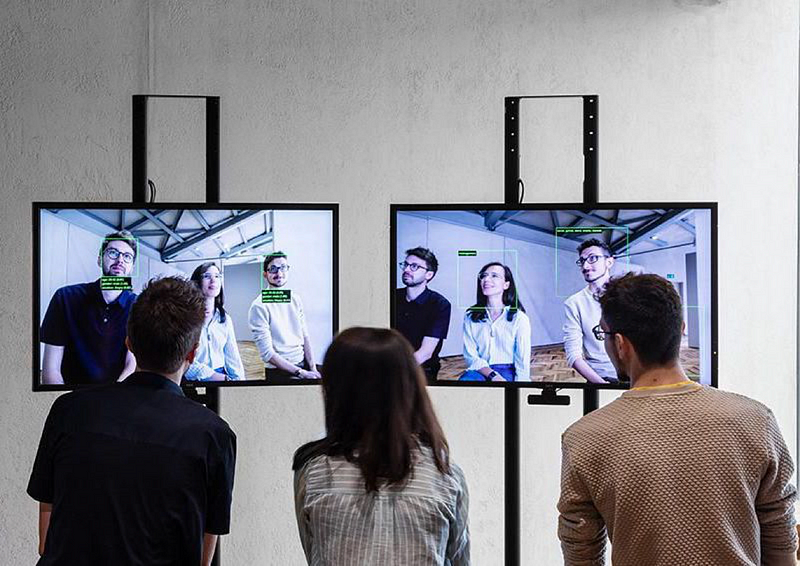
ImageNet Roulette is a video installation and app that accompanies their exhibition.
It went viral in September, after millions of people uploaded their photos to see how they would be classified by ImageNet. This is a question with significant implications. ImageNet is the canonical object recognition dataset. It has done more than any other to shape the AI industry.
While some of ImageNet’s categories are strange, or even funny, the dataset is also filled with extremely problematic classifications, many of them racist and misogynist. Imagenet Roulette provided an interface for people to see into how AI systems classify them — exposing the thin and highly stereotyped categories they apply to our complex and dynamic world. Crawford and Paglen published an investigative article revealing how they opened up the hood on multiple benchmark training sets to reveal their political structures.
This is another reason why art and research together can sometimes have more impact than either alone, making us consider who gets to define the categories into which we are placed, and with what consequences.
3. CITIES, SURVEILLANCE, BORDERS

Issues of power, classification, and control are at the foreground of the large scale roll-out of corporate surveillance systems across the U.S this year. Take Amazon’s Ring, a surveillance video camera and doorbell system designed so people can have 24/7 footage of their homes and neighborhoods.
Amazon is partnering with over 400 police departments to promote Ring, asking police to convince residents to buy the system. A little like turning cops into door-to-door surveillance salespeople.
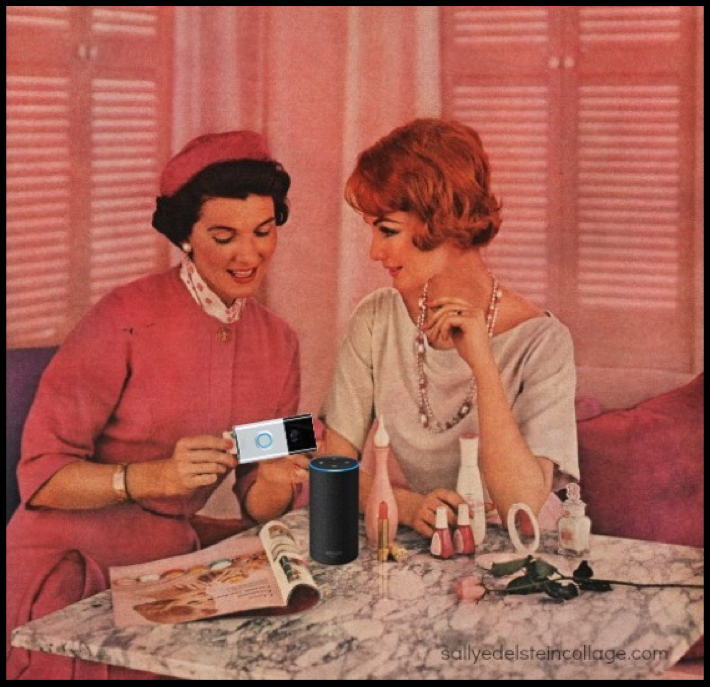
As part of the deal, Amazon gets ongoing access to video footage; police get access to a portal of Ring videos that they can use whenever they want. The company has already filed a patent for facial recognition in this space, indicating that they would like the ability to compare subjects on camera with a “database of suspicious persons” — effectively creating a privatized surveillance system of homes across the country.
But Ring is just one part of a much bigger problem. As academics like Burku Baykurt, Molly Sauter and AI Now fellow Ben Green have shown, the techno-utopian rhetoric of “smart cities” is hiding deeper issues of injustice and inequality.
And communities are taking this issue on. In August, San Diego residents protested the installation of “smart” light poles, at the exact same time as Hong Kong protesters were pulling down similar light posts and using lasers and gas masks to confound surveillance cameras.
And in June this year, students and parents in Lockport NY protested against a facial recognition system in their school that would give the district the ability to track and map any student or teacher at any time. The district has now paused the effort.
Building on the fights against tech companies reshaping cities, from Sidewalk Toronto’s waterfront project to Google’s expansion into San Jose, researchers and activists are showing the connections between tech infrastructure and gentrification, something that the Anti Eviction Mapping project, led by AI Now postdoc Erin Mcelroy, has been documenting for years.
And of course, in February a large coalition here in New York pushed Amazon to abandon its second headquarters in Queens. Organizers highlighted not only the massive incentive package New York had offered the company, but Amazon’s labor practices, deployment of facial recognition, and contracts with ICE. It’s another reminder of why these are multi-issue campaigns — particularly given that tech companies have interests across so many sectors.
Of course, one of the most unaccountable and abusive contexts for these tools is at the US southern border, where AI systems are being deployed by ICE and Customs and Border Patrol.
Right now there are 52,000 immigrants confined in jails, prisons, and other forms of detention, and another 40,000 homeless on the Mexico side of the border, waiting to make an asylum case. So far, seven children have died in ICE custody in the past year, and many face inadequate food and medical care. It’s hard to overstate the horrors happening right now.
Thanks to a major report by the advocacy organization Mijente, we know that companies like Amazon and Palantir are providing the engine of ICE’s deportations. But people are pushing back — already, over 2,000 students across dozens of universities have signed a pledge not to work with Palantir, and there have been near weekly protests at the head offices of tech companies contracting with ICE.
We are honored to be joined tonight by Mijente’s executive director, Marisa Franco, who was behind this report and a leader in the “NoTechForIce” movement.
4. LABOR, WORKER ORGANIZING and AI
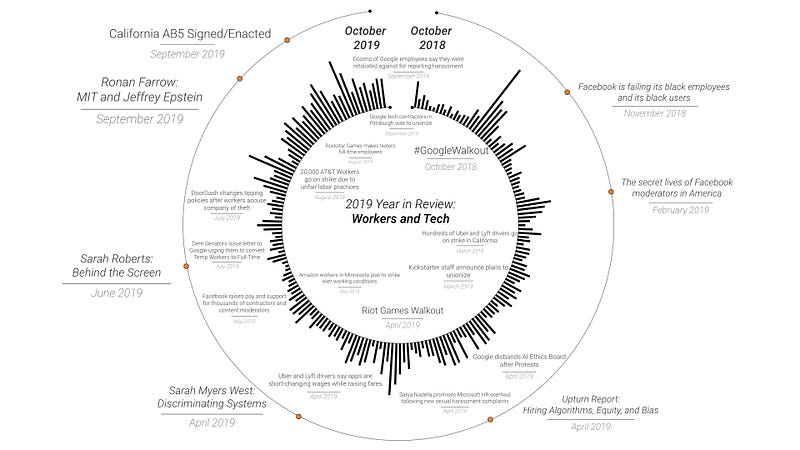
Of course, problems with structural discrimination along lines of race, class, and gender are on full display when we examine the AI field’s growing diversity problem.
In April, AI Now published Discriminating Systems, led by AI Now postdoc Sarah Myers West. This research showed a feedback loop between the discriminatory cultures within AI, and the bias and skews embedded in AI systems. The findings were alarming. Just as the AI industry established itself as a nexus of wealth and power, it became more homogenous. There is clearly a pervasive problem across the field.
But there are also growing calls for change. Whistleblower Signe Swenson and journalist Ronan Farrow helped reveal a fundraising culture at MIT that put status and money above the safety of women and girls. One of the first people to call for accountability was Kenyan Graduate Student, Arwa Mboya. Her call for justice fit a familiar pattern in which women of color without much institutional power are the first to speak up. But of course MIT is not alone.
We’ve seen a series of walkouts and protests across multiple tech companies, from the Google Walkout, to Riot Games, to Microsoft workers confronting their CEO, all demanding an end to racial and gender inequity at work.
Now, as you may have heard, AI Now Co-founder Meredith Whittaker left Google earlier this year. She was increasingly alarmed by the direction of the industry. Things were getting worse, not better, and the stakes were extremely high. So her and her colleagues started organizing around harmful uses of AI and abuses in the workplace, taking a page from teacher’s unions and others who’ve used their collective power to bargain for the common good.
This organizing work was also informed by AI Now’s research and the scholarship of so many others, which served as an invaluable guide for political action and organizing. Along the way the tech worker movement grew, there were some major wins, and some experiences that highlighted the kind of opposition those who speak up often face.
Contract workers are a crucial part of this story. They were some of the first to organize in tech, and carved the path. They make up more than half the workforce at many tech companies, and they don’t get the full protections of employment, often earning barely enough to get by, and laboring on the sidelines of the industry. Work from scholars like Lilly Irani, Sarah Roberts, Jessica Bruder, and Mary Gray, among others helped draw attention to these shadow workforces.
AI platforms used for worker management are also a growing problem. From Uber to Amazon warehouses, these massive automated platforms direct worker behavior, set performance targets, and determine workers wages, giving workers very little control.
For example, earlier this year, Uber slashed worker pay without explanation or warning, quietly implementing the change via an update to their platform. Meanwhile drivers for delivery company Door Dash revealed that the company was — quite literally — stealing the tips customers thought they left them in the app.
Happily, we’ve also seen some big wins for these same workers. Rideshare workers in CA had an enormous victory with the AB-5 law, which requires app-based companies to provide drivers the full protections of employment. It’s a monumental change from the status quo, and to discuss this significant moment, Veena Dubal is joining us tonight from UC Hastings. She’s a leading academic studying the gig economy, and she’s worked with drivers and activists for years.
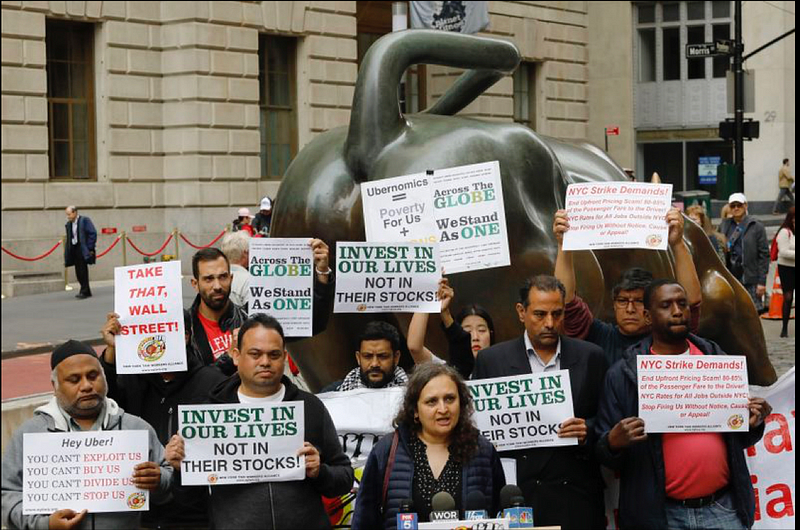
On the east coast, Bhairavi Desai heads the New York Taxi Workers Alliance, a union she founded in 1998 that now has over 21,000 members. Bhairavi led one of the first campaigns to win against rideshare companies, and she’s with us tonight to discuss that work.
Finally, we’re honored to have Abdi Muse on the same panel. He’s the Executive Director of the Awood Center outside of Minneapolis, and a veteran labor organizer who worked with Amazon Warehouse workers in his community to bring the famously resistant company to the table and get concessions that improved their lives. Getting Amazon to do anything is a major feat, and this was a first.
AI’S CLIMATE IMPACT
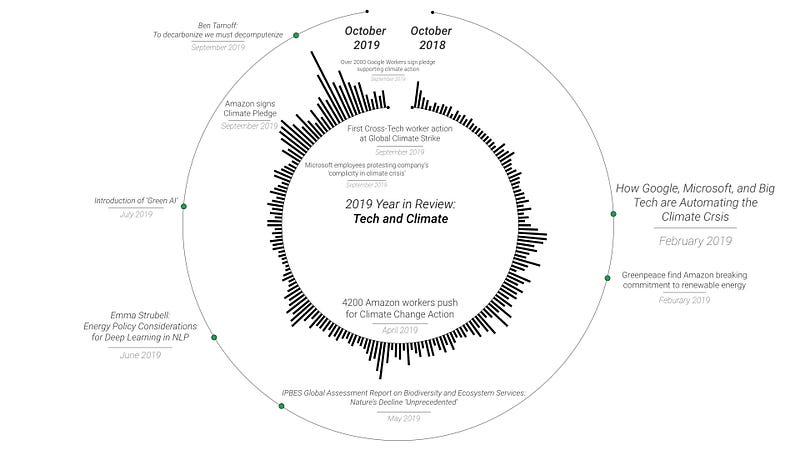
The backdrop to all these issues is the climate. Planetary computation is having planetary impacts.
AI is extremely energy intensive, and uses a large amount of natural resources. Researcher Emma Strubell from Amherst released a paper earlier this year that revealed the massive carbon footprint of training an AI system. Her team showed that creating just one AI model for natural-language processing can emit as much as 600,000 pounds of carbon dioxide.
That’s about the same amount produced by 125 roundtrip flights between New York and Beijing.
The carbon footprint of large-scale AI is often hidden behind abstractions like “the cloud.” In reality, the world’s computational infrastructure is currently estimated to emit as much carbon as the aviation industry, a dramatic percentage of global emissions. But here too, there is growing opposition. Just this month we saw the first ever cross-tech sector worker action — where tech workers committed to strike for the climate.
They demanded zero carbon emissions from big tech by 2030, zero contracts with fossil fuel companies, and for companies not to deploy their technology to harms climate refugees. Here we see the shared concerns between the use of AI at the borders, and the movement for climate justice. These are deeply interconnected issues — as we’ll be seeing on stage tonight. So let’s close it out by looking at the full visual timeline of the year, that brings all these themes together.
THE GROWING PUSHBACK
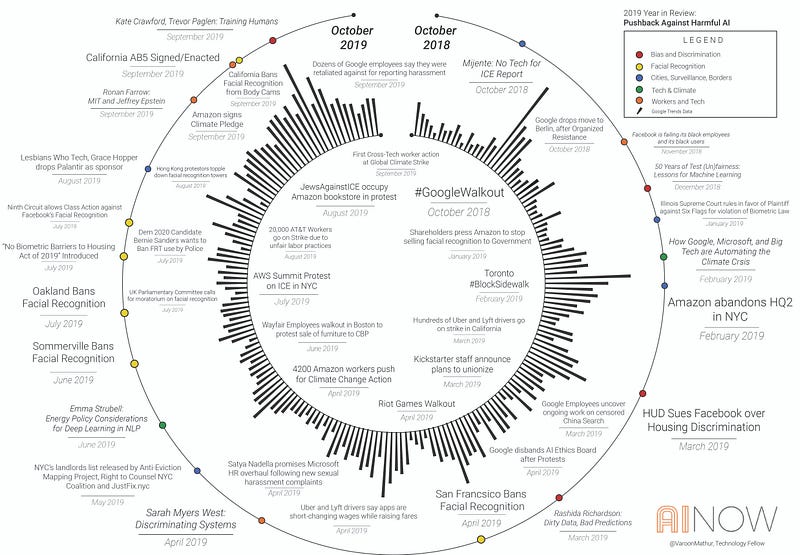
You can see there’s a growing wave of pushback emerging. From rejecting the idea that facial recognition is inevitable, to tracing tech power across spaces in our homes and our cities, an enormous amount of significant work is underway.
And it’s clear that the problems raised by AI are social, cultural, and political — as opposed to primarily technical. These issues, from criminal justice, to worker rights, to racial and gender equity, have a long and unbroken history. Which means that those of us concerned with the implications of AI need to seek out and amplify the people already doing the work, and learn the histories of those who’ve led the way. These are the people you’ll hear from tonight.
The pushback that defined 2019 reminds us that there is still a window of opportunity to decide what types of AI are acceptable and how to make them accountable. Those on stage this evening are on the front lines of creating this real change — researching, organizing and pushing back, across multiple domains.
They share a common commitment to justice, and a willingness to look beyond the hype, and to ask who benefits from AI, who is harmed, and who gets to decide.
The organizing, legislation, and scholarship represented in the visual timeline capture some of the key moments in pushback against harmful AI and unaccountable tech power over the last year. This is not an exhaustive list, but a snapshot of some of the ways workers, organizers, and researchers are actively resisting. We used a circular framework, placing grassroots campaigns and labour organizing driven by tech workers and community members at the center of the visualization. Significant events and publications that reflected, responded to, or provided analysis of core issues related to this pushback are represented in the outer circles. The bars along the circumference of the inner circle reflect Google trend data points for each of the events — visualizing one measure of how these campaigns and initiatives have gained attention online.