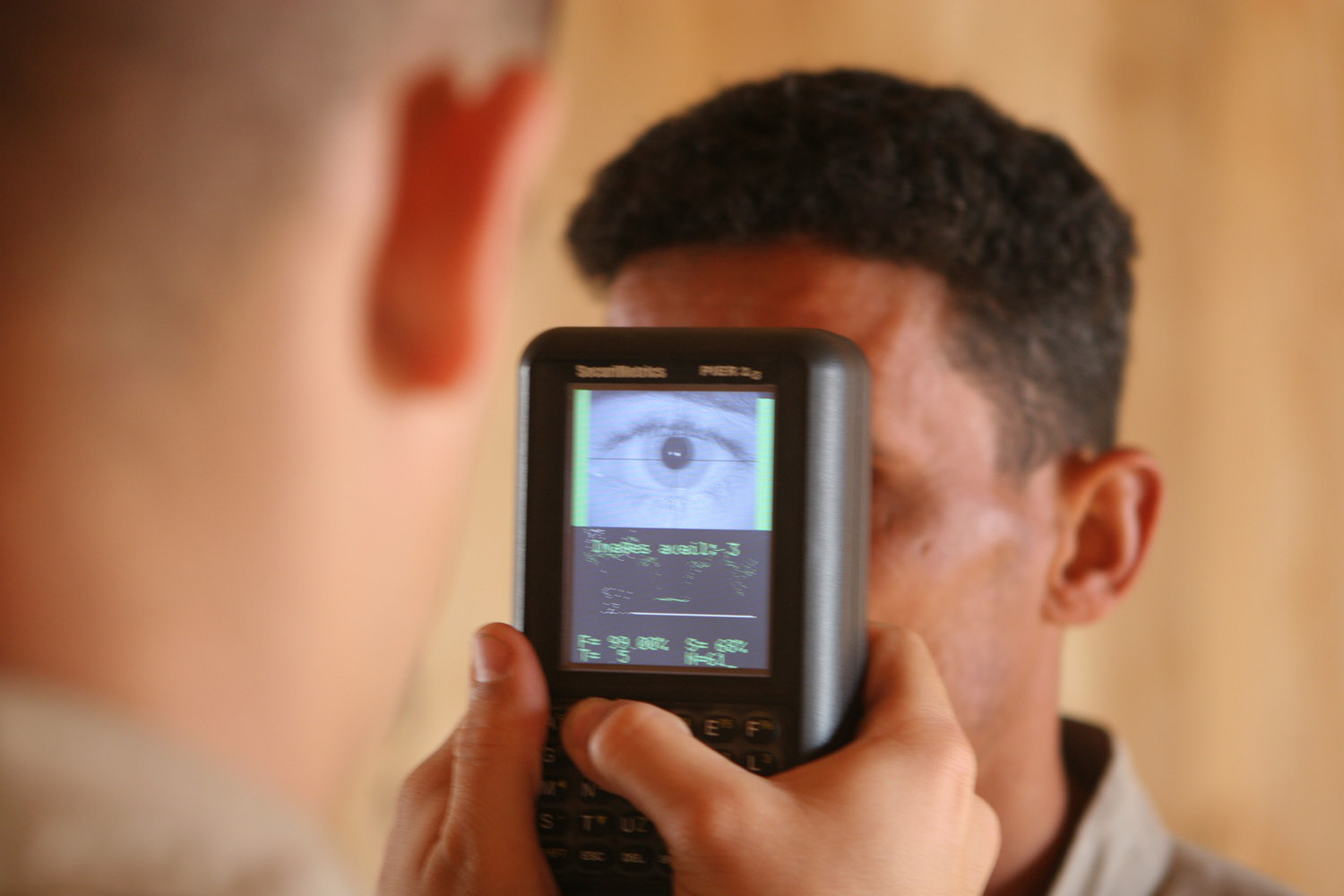
Sociotechnical Misalignments in
AI-Mediated Behavioral Health
The application of artificial intelligence (AI) to the behavioral health domain has led to a growing interest in the use of machine learning (ML) techniques to identify patterns in people’s personal data with the goal of detecting-and even predicting-conditions such as depression, bipolar disorder, and schizophrenia. This paper investigates the data science practices and design narratives that underlie AI-mediated behavioral health through the situational analysis of three natural language processing (NLP) training datasets. Examining datasets as a sociotechnical system inextricably connected to particular social worlds, discourses, and infrastructural arrangements, we identify several misalignments between the technical project of dataset construction and benchmarking (a current focus of AI research in the behavioral health domain) and the social complexity of behavioral health.