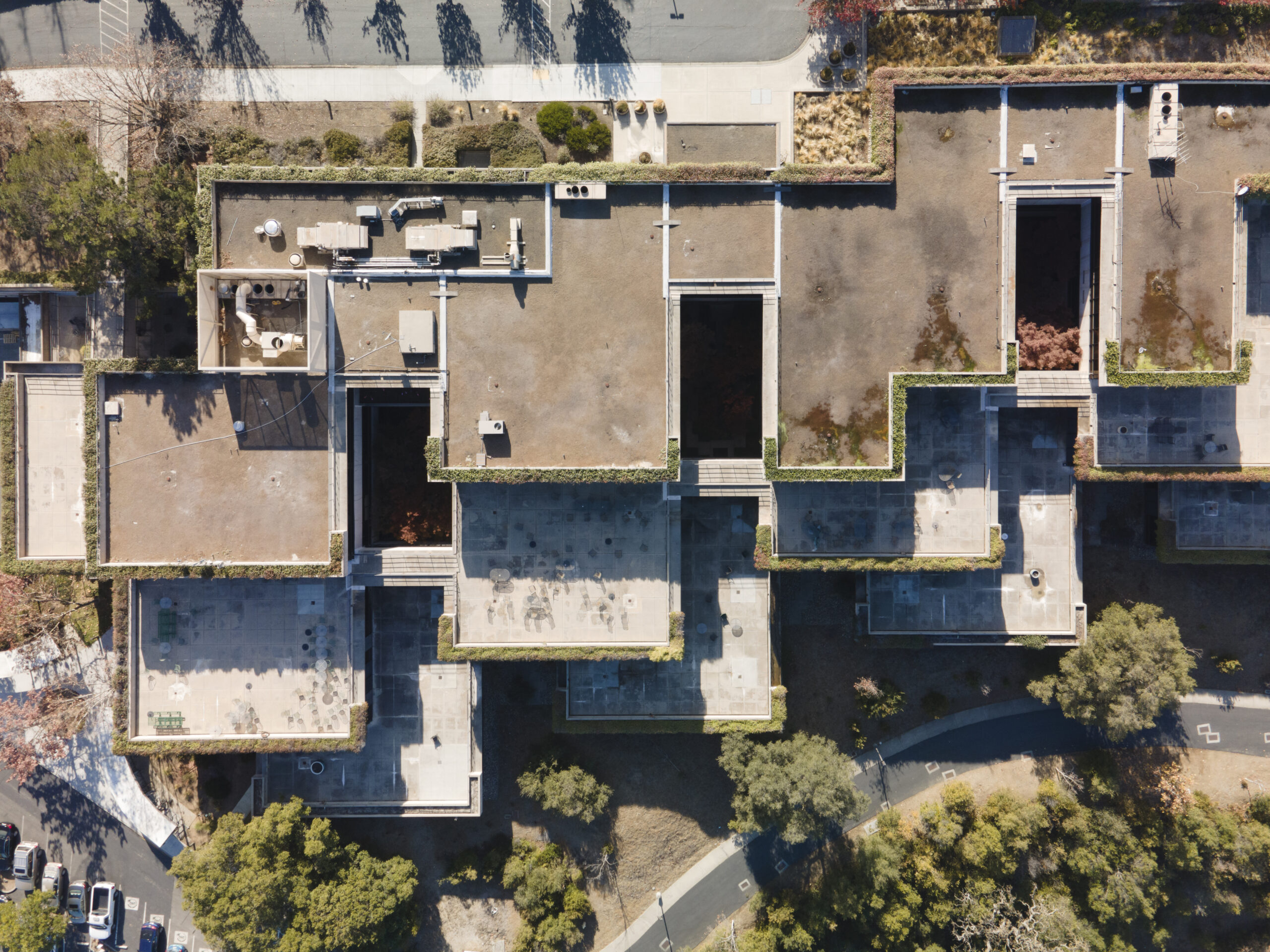
AI Now Institute Co-Executive Director Amba Kak testified at a Senate Committee on Commerce, Science and Transportation Hearing on “The Need to Protect Americans’ Privacy and the AI Accelerant“. Read her testimony here.
—————
Chair Cantwell, Ranking Member Cruz, and esteemed Members of the Committee, thank you for inviting me to testify.
We’re at a clear inflection point in the trajectory of AI. Without guardrails to set the rules of the road, we’re committing ourselves to carrying forward more of the same: extractive, invasive, and often predatory data practices and business models that characterized the past decade of the tech industry.
We’re committing ourselves to the seamless transition of Big Tech from surveillance monopolies to AI monopolies. We must break this cycle.
A federal data privacy law, especially one with strong data minimization, could challenge the culture of impunity and recklessness in the AI industry that’s already hurting both consumers and competition. If there’s any single point I want to make today: it’s that now is the moment where passing such a law matters most: before the trajectory has been set.
Data privacy regulation is AI regulation and it provides many of the essential tools that we need to protect the public from harm. So let’s start there: how might the AI market shape up differently in the presence of a strong data privacy law?
First, with data minimization rules: firms will need to put reason in place of recklessness when it comes to decisions about what data to collect, the purposes to which it can be used, and for how long it should be stored. Such requirements would empower lawmakers – and the public – to demand basic accountability.
Before Microsoft rushes to release its Recall AI feature – that takes continuous screenshots of everything you see or do on your computer – the company need to ask itself AND document the answer: does the utility of this feature outweigh the honey pot its creating for bad actors? As a security researcher quickly discovered, with Recall, it’s scarily trivial for an attacker to use malware to extract a record of everything you’ve ever viewed on your PC. A strong data minimization mandate would have nipped this in the bud: likely disincentivized the development of such a patently insecure feature to begin with.
Second, we’d have basic transparency about the data decisions that affect us all. Meta and Google recently announced updates to their terms that explicitly allow AI training from user data. We know about this because European users were alerted by Meta: without a legal mandate to require it, American users received no such notification. Users of Reddit are also up in arms, because their content was just sold to the highest bidder – Google – for use in training its AI.
Now a privacy law would offer more than just transparency in these isntances: purpose limitation rules would prevent Big Tech from using AI as its catch all justification to use and combine data across contexts and store it forever. The FTC has already penalized Amazon for storing children’s voice data indefinitely using AI as its excuse. But we can’t rely on this kind of one off enforcement, these need to be the rules of the road.
This moves would not only safeguard our privacy, they would also act as a powerful check on the data advantages currently being consolidated by Big Tech to stave off competition in AI.
Third, in a world with a data privacy mandate, AI developers would need to make data choices that deliberately prevent discriminatory outcomes. We shouldn’t be surprised when women see far less ads for high paying jobs on Google Ads, that’s 100% a feature of data decisions made upstream. These are avoidable problesm, and it’s not just in scope for a data privacy law, it’s integral to protecting people from the most serious abuses of our data. And where specific AI practices have inherent well established harms, so-called “emotion recognition” systems that lack any scientific validity, or pernicious forms of targeted ads, the law could hold them entirely offlimits.
Finally, and here’s the thing about large-scale AI: it is not only computationally, ecologically, and data intensive, it is also very, very expensive to develop and run these systems. These eye-watering costs will need a path to profit. By all accounts, though, a viable business model still remains elusive. It is precisely in this kind of environment, with a few incumbent firms feeling the pressure to turn a profit, that predatory business models tend to emerge.
Meanwhile, new research suggests LLMs are capable of hyper personalized inferences about us even from the most general prompts. You don’t need to be clairvoyant to see that all roads might well be leading us right back to the surveillance advertising business model, even for generative AI, with all its attendant pathologies.
To conclude, there is nothing about the current trajectory of AI that is inevitable. As a democracy, the US has the opportunity to take global leadership in shaping this next era of tech so that it reflects public interest, not just the bottom lines of a handful of companies. This is a moment for action.